
Financial institutions are facing a relentless rise in fraud. USD 1.03 trillion was lost to scams worldwide in 2024. Fraud is also becoming more sophisticated. It has evolved from account takeover scams to complex AI fraud. The rise of platforms like OnlyFake and deepfake scams can attest to that fact.
Fraudsters are also using advanced technologies in existing scams. For instance, they may use deepfakes to control someone’s bank account.
Since the fraud attempts are sophisticated, they require a solution that is equally, if not more, sophisticated. AI and machine learning are helping companies become more proactive in catching fraud by highlighting attributes that may not be apparent but indicate a much larger pattern of fraud.
These systems combine machine learning models, rule-based logic, real-time data monitoring, and anomaly detection to identify suspicious activities that are often invisible to manual review.
How AI Agents Detect Fraud
AI systems employ multiple techniques in tandem. Consider combining anomaly detection, risk scoring, network analysis of relationships, text mining for fraud signals, identity verification, and more.
Machine Learning Models vs. Rule-Based Systems:
Modern fraud detection heavily relies on machine learning models that learn complex patterns from data, far surpassing static if-then rules.
Traditional rule-based systems (e.g. “flag any transaction over $5,000 from a new location”) are simple to implement but often rigid and prone to high false positives. They can’t easily capture nonlinear interactions between factors or adapt to new fraud tactics.
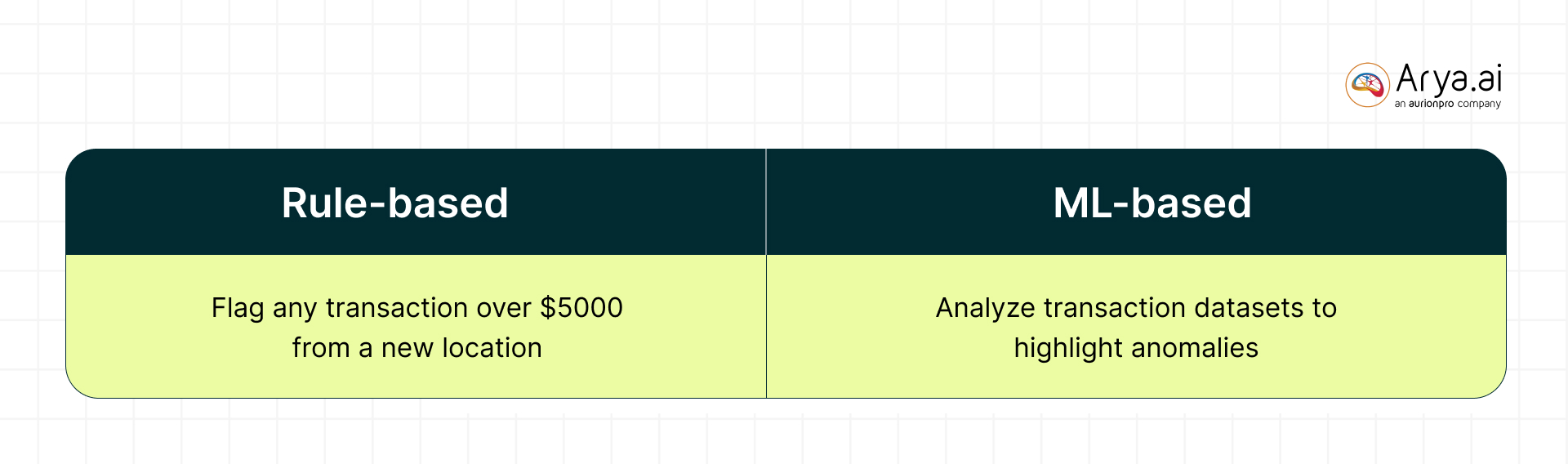
ML models, by contrast, can analyze vast transaction datasets to recognize subtle correlations and unusual combinations of features that indicate fraud.
For example, supervised ML algorithms can be trained on labeled historical transactions (fraudulent vs. legitimate) to predict fraud risk. Unsupervised models can detect outliers that deviate from normal behavior
FIs typically go with a hybrid approach. Well-defined rules help catch known fraud patterns, and an ML-based risk-scoring model handles more complex, evolving patterns.
Real-Time Monitoring and Anomaly Detection
Transactions are monitored in real time and scored on the fly, so potentially fraudulent payments can be blocked or reviewed before completion.
Banks deploy models within streaming pipelines or use fast in-memory computing to enable this. Each incoming transaction is compared against a customer’s typical behavior profile and known fraud indicators (e.g. abnormal amount, new device or location, rapid-fire transfers).
If a transaction deviates significantly from the norm, the system flags it for immediate investigation or automated countermeasure. This real-time, adaptive monitoring marks a leap from older systems that often reviewed transactions in batches after the fact.
Pattern Recognition and Network Analysis
Clustering and graph-based machine learning can identify networks of linked entities. In insurance, AI may detect a ring of claimants, doctors, and vehicles interlinked by common details (addresses, phone numbers, IP addresses). This highlights red flags that indicate organized fraud. Similarly, in banking, network analysis can uncover mule account networks.
These AI tools map relationships and find communities or sequences that a human investigator might miss. Such link analysis helps detect complex schemes like money laundering chains or staged accident rings. AI models also apply text analysis to unstructured data, scanning emails, claims descriptions, or call transcripts for keywords or linguistic patterns indicative of fraud (e.g., identical phrasing in multiple claims). Moreover, identity verification algorithms use ML for document recognition and face matching to spot fake IDs or impersonation attempts.
All these components – anomaly flags, risk scores, network linkages, and text signals – are often combined to give a holistic fraud risk assessment for each event or entity.
Continuous Learning and Model Update
Fraudsters constantly tweak their methods, so AI fraud detection must evolve accordingly. Advanced systems employ adaptive learning, retraining models on newly confirmed fraud cases, and updated customer behavior.
Many institutions periodically refresh their ML models with recent data, and some use online learning or reinforcement learning to adjust thresholds on the fly.
If criminals start testing stolen cards with small transactions to avoid detection, an adaptive model can learn this emerging pattern and tighten sensitivity for such sequences. This ability to “learn from experience” in near real-time enables AI to catch novel fraud tactics that were not explicitly programmed into the system.
It also helps reduce false positives over time—the model refines what is genuinely suspicious versus a benign anomaly, improving accuracy as it ingests more outcomes. In short, AI agents bring a dynamic, data-driven defense that grows more effective with each fraud attempt thwarted, whereas static rules would require manual updating and still lag behind new schemes.
Where Will These AI Systems Be Useful
AI agents are being applied across various fraud scenarios in banking and insurance. Here are a few:
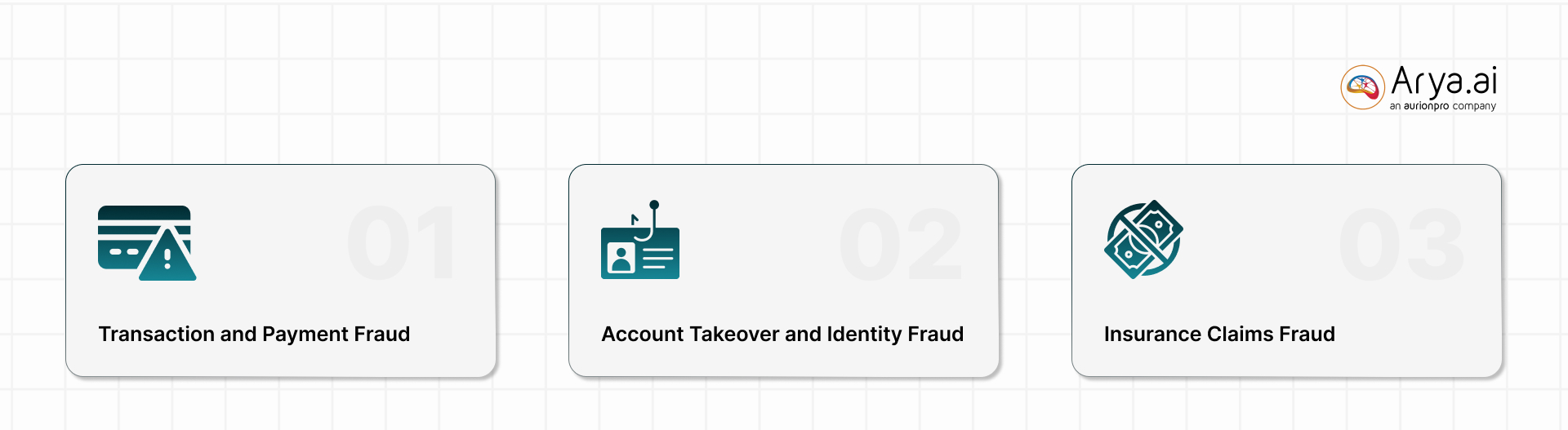
Transaction and Payment Fraud
One of the most common challenges is detecting fraudulent transactions in real time. The speed and volume of credit/debit card fraud and unauthorized transfers overwhelm the systems and workforce.
AI models analyze attributes of each payment – amount, time, merchant, geolocation, device ID, spending pattern, etc. – to spot anomalies that suggest fraud.
If a credit cardholder who usually spends only in one city suddenly makes purchases overseas quickly, an ML model would flag it as high-risk. Similarly, multiple rapid transactions or a large purchase outside a customer’s normal range can trigger the AI’s suspicion.
Banks use these risk scores to automatically decline a transaction or step up authentication (like sending a one-time passcode) before allowing it. AI-based transaction monitoring has significantly improved detection rates for payment fraud.
Visa, for instance, uses real-time deep learning models in its authorization network and reported that its system (Visa Advanced Authorization) helped prevent an estimated $27 billion in attempted fraud in 2022 alone.
Account Takeover and Identity Fraud
Account takeover (ATO) fraud occurs when a bad actor gains illicit access to a user’s bank account, credit card account, or online banking profile and then attempts unauthorized transactions or changes.
They can analyze login attempts (device used, IP address location, time of access, typing rhythm, etc.) and compare them to the genuine user’s standard profile.
Unusual access – like an account accessed from a new country and device after multiple failed logins – would be flagged as a potential takeover.
Similarly, if a user typically logs in once a week but suddenly there’s a burst of logins and fund transfers, an AI system would catch that behavioral anomaly. Many banks also deploy continuous authentication powered by AI, which scores session behaviors (navigation habits, mouse movements, etc.) to ensure the account user is a legitimate customer. In addition, AI-driven identity verification is used during onboarding or password resets: computer vision can validate ID documents and selfies to prevent fraudsters from using stolen personal data to open fake accounts or reset someone’s password.
These AI defences are crucial with phishing and credential-stuffing attacks on the rise. They often work hand-in-hand with cybersecurity systems – for example, if a known data breach might expose user credentials, AI models raise vigilance for those accounts. By catching account takeovers early (sometimes after one suspicious transaction), banks can lock the account and alert the customer, minimizing losses and damage.
Insurance Claims Fraud
Claims fraud can take many forms: exaggerated claims, entirely fake claims, staged accidents, repeated claims for the same damage, and more. Traditionally, insurers relied on human claim investigators to spot red flags, which is less and less viable daily.
Machine learning models analyze claim data (submitted documents, photos, claim history, policy details, etc.) and look for inconsistencies or outliers. For instance, an AI model might flag an auto insurance claim where repair costs significantly exceed the norm for the reported damage or a health insurance claim showing treatments that don’t match the diagnosed injury.
Anomaly detection is applied to things like the timing and frequency of claims. The system will notice if multiple claims come from the same person or clinic quickly or if a new claimant has a pattern matching known fraudulent profiles. Some insurers use natural language processing to read descriptions in claim forms and compare them to past fraudulent narratives.
This has streamlined their process: honest customers get paid faster, and fraudulent payouts are halted. Given that 10-20% of insurance claims are fraudulent, AI tools boost the fight against bogus claims.
Real-World Scenario
Many financial institutions and insurers have reported significant improvements after implementing AI-driven fraud detection systems. HSBC built an AI-based “Dynamic Risk Assessment” system to screen transactions for financial crime.
In a live pilot, the bank found 2–4× more suspicious activity than its previous system, cutting false positives (legitimate transactions flagged in error) by 60%. This AI system now analyzes 1.35 billion monthly transactions across 40 million accounts, slashing the review processing time from weeks to days.
Replacing its rule-based fraud engine with an advanced machine learning one, Danske Bank achieved a 60% reduction in false positives (to reach 80%) and a 50% increase in true fraud detection. This saved millions in fraud losses and investigation costs.
Mastercard developed a generative AI system to identify compromised cards and fraudulent transactions across its network. In 2024, they announced that this technology is doubling the detection rate of card compromises (catching breached card details faster) and reducing false declines by up to 200% (i.e., cutting false positives to a fraction of previous levels). It also triples the speed of spotting at-risk merchants.
A common theme across the examples is the reduction of false positives (legitimate customer actions incorrectly tagged as fraud) when using AI. This is a huge benefit and a much better experience for customers. It proves that AI is catching fraud and making fraud operations more efficient.
Human–AI Collaboration and System Integration
The sophistication of AI systems does not sideline human expertise and oversight. The interaction between AI systems and human fraud analysts, often called a “human-in-the-loop” approach, ensures optimal results.
In practice, AI-driven fraud systems work alongside human analysts. Autonomous finance systems monitor incoming data and flag anomalies or high-risk events in real time, as seen on the analyst’s dashboards above. Human experts then review these AI-generated alerts to confirm actual fraud cases, investigate complex incidents, and continuously refine the AI’s performance.
In most deployments, the AI’s role is to sift through massive volumes of transactions or claims and raise alerts on the few that look suspicious. These alerts are then given to human fraud investigators or analysts for validation. This workflow capitalizes on each's strengths—the AI quickly combs data and detects patterns, while humans apply judgment and context to confirm fraud and filter out any false alarms that slip through.
For example, an AI might flag a customer’s unusual credit card purchase in a new city. Still, a human analyst (or an automated second-factor query to the customer) can verify whether the customer is travelling or the charge is unauthorized. This collaborative process dramatically speeds up fraud response.
AI reduces the caseload on investigators by only forwarding high-risk cases, and those analysts, in turn, provide feedback that helps improve the models (such as labeling an alert as false so the AI learns from that mistake). Over time, the AI’s precision improves, but a human check is still essential, especially for borderline cases and complex fraud rings that may require deeper investigation.
Conclusion
AI is indispensable in the fight against fraud. It excels at real-time monitoring, analyzing each transaction and claim against learned patterns and flagging the slightest hint of anomaly. AI can prevent credit card and payment fraud, account takeovers, and the sniffing of bogus insurance claims. Delivering tangible results, AI is registering higher fraud detection rates, fewer false alarms, and faster investigation cycles. Banks and FIs are saving millions that could have been lost to fraud. HSBC, JPMorgan, and Visa have all reported significant gains in efficiency and accuracy.
Rather than replacing human fraud experts, AI works alongside them, handling the heavy data lifting and preliminary analysis so that human investigators can apply their judgment to a refined set of high-quality alerts. This synergy between AI and humans leverages the best of both: the speed and pattern recognition of machines with the intuition and contextual understanding of people.
As fraudsters adopt new tricks (even using AI themselves to probe systems or craft deep fake identities), the fraud detection landscape will continue to evolve. If you’d like to arrest fraud in its tracks, connect with Arya.ai today. We have built production-ready AI solutions to help deal with the evolving risk landscape and prevent fraud.
Book a meeting with Arya.ai today.