.jpg)
Mule accounts allow fraudsters to move illegal funds through the banking system without raising suspicion. In India alone, mule accounts are responsible for more than half of all financial fraud cases, with nine out of ten cases going undetected.
Banks freeze hundreds of thousands of these accounts every year. And yet, fraudsters quickly create new accounts, making it a never-ending cycle. Faster and more effective detection methods are needed urgently. Leveraging AI to automate mule account detection can help.
In this blog, we’ll break down the risks that mule accounts pose to banks, why traditional detection methods are no longer enough, and why we need AI-driven solutions.
Different Types of Mule Accounts
Mule accounts are bank accounts that are set up by fraudsters or unwitting intermediaries, commonly known as "money mules," to transfer money obtained from illegal activities. These accounts obscure the true origin of funds, making it difficult to trace money laundering and fraud.
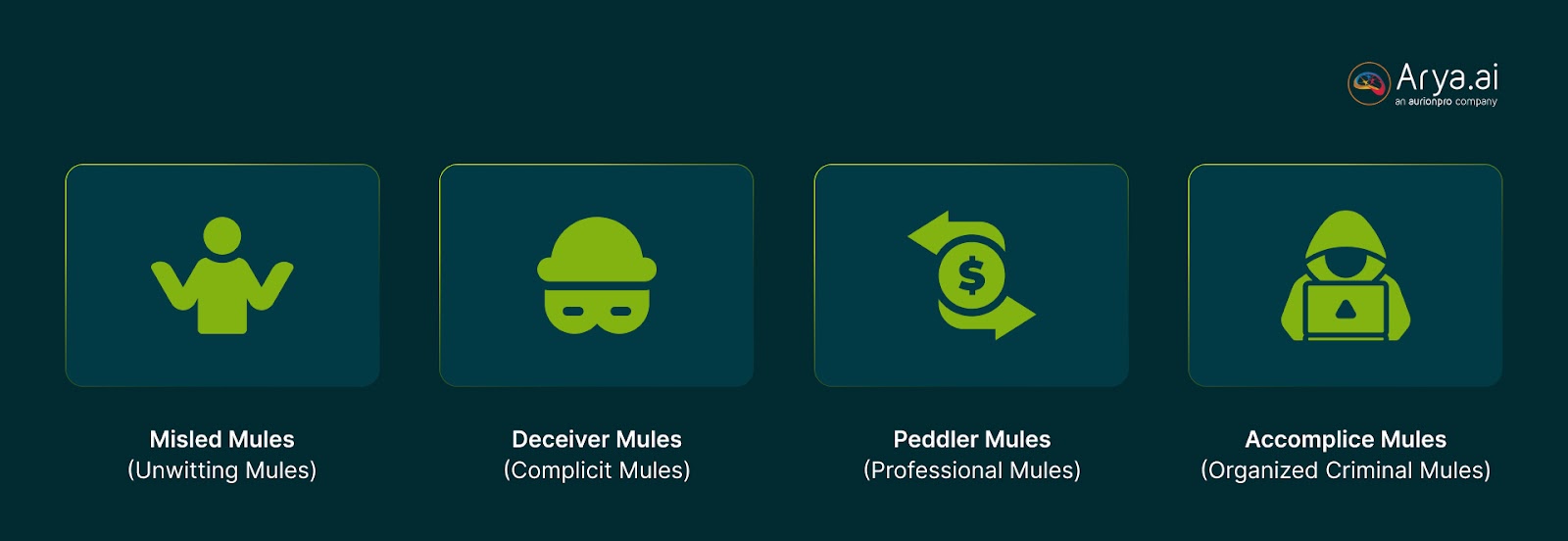
Here are the different types of mule accounts you should know about:
- Misled Mules (Unwitting Mules): These individuals are unaware they are being used for fraud, making them harder to detect using traditional rule-based systems
- Deceiver Mules (Complicit Mules): Deceiver mules are aware of their involvement in fraudulent activities but are convinced they won’t face legal consequences.
- Peddler Mules (Professional Mules): These individuals operate mule accounts deliberately as a source of income. They may own or control multiple bank accounts and actively work with fraud networks to move illicit funds. Peddler mules may also recruit others into the scheme, expanding fraudulent activities across multiple jurisdictions.
- Accomplice Mules (Organized Criminal Mules): These are high-level players in organized financial crime networks. They don’t just move money, they actively assist in fraud planning, documentation forging, and laundering large-scale illicit proceeds.
Mule Accounts: More Than a Nuisance for Financial Institutions
Mule accounts are not just minor inconveniences. They pose a severe systemic threat. These accounts, whether operated by willing participants or unsuspecting individuals, create systemic vulnerabilities that threaten the integrity of banks and financial markets.
The National Crime Records Bureau (NCRB) data revealed that online financial frauds constitute 67.8% of all cybercrime complaints received in Q2 2022. A significant challenge in preventing financial fraud is the use of money mule accounts.
According to RBI's Innovation Hub website, online financial fraud accounted for 67.8% of all cyber crime complaints in Q2 2022. Over 450,000 mule accounts were frozen in just one year, underscoring the global scale of the problem.
Here’s why mule accounts are a threat to banks and financial institutions;
1. Systemic Vulnerability in Financial Systems
Mule accounts are often used to launder money and facilitate fraud. When left unchecked, they erode trust in banks, expose institutions to regulatory fines, and distort financial data, leading to poor credit assessments.
2. Aggressive Growth Targets and lax Verification
Banks often set high targets for opening new accounts, putting pressure on employees to meet them quickly. In an attempt to meet these targets, bank executives often overlook using rigorous verification processes. This weakens strict KYC (Know Your Customer) checks and creates opportunities for fraudsters to open mule accounts undetected.
3. Unwitting Participation of Customers
Many people unknowingly become money mules when fraudsters trick them into using their bank accounts to move illegal money. They might be promised easy earnings, asked to help a "friend," or offered jobs that involve receiving and transferring funds. Without realizing it, they assist in fraud and money laundering, putting themselves at serious legal and financial risk.
4. Limitations of Traditional Detection Systems
Conventional systems are ill-equipped to detect the subtle and evolving tactics of modern fraudsters. Their inability to adapt to new patterns results in high false negatives and missed fraudulent activities.
5. Fragmented Verification Practices Across Institutions
Inconsistencies in verification processes among financial institutions create exploitable gaps. A lack of standardized, cross-industry protocols allows mule accounts to be established and maintained undetected, as fraudsters exploit the disjointed nature of current systems.
Why We Should Look Beyond Traditional Solutions
Traditional methods for detecting mule accounts rely on rule-based monitoring, manual KYC checks, and pattern recognition to flag suspicious activity. Banks set transaction limits, track irregular fund transfers, and verify identities through official documentation.
These methods are becoming less effective because fraudsters have found new ways to avoid detection. They now use fake identities, break large transactions into smaller ones (structured transactions), and spread their activities across multiple banks.
For example, instead of depositing $50,000 at once, an amount that might attract scrutiny, a fraudster might deposit $9,900 across multiple accounts to stay below reporting thresholds. This tactic helps criminals move illicit funds through the banking system without being detected.
Traditional detection methods have proven inadequate in identifying these sophisticated schemes, necessitating the adoption of advanced AI solutions. Manual investigations and watchlist screenings can only do so much. By replacing static rule-based monitoring with intelligent AI models, banks can better identify, track, and prevent mule account activity before fraud occurs.
How to Detect Mule Accounts Using AI
AI-powered solutions are capable of proactively detecting and shutting down mule accounts before they cause financial and reputational damage. They do this by analyzing transaction patterns, behavioral biometrics, and anomalies in real-time.
In India, the RBI has stepped up to ensure that mule account activity can be easily detected. The RBI's Reserve Bank Innovation Hub has introduced MuleHunter AI — an advanced AI/ML-based system designed to effectively detect and neutralize mule account activity.
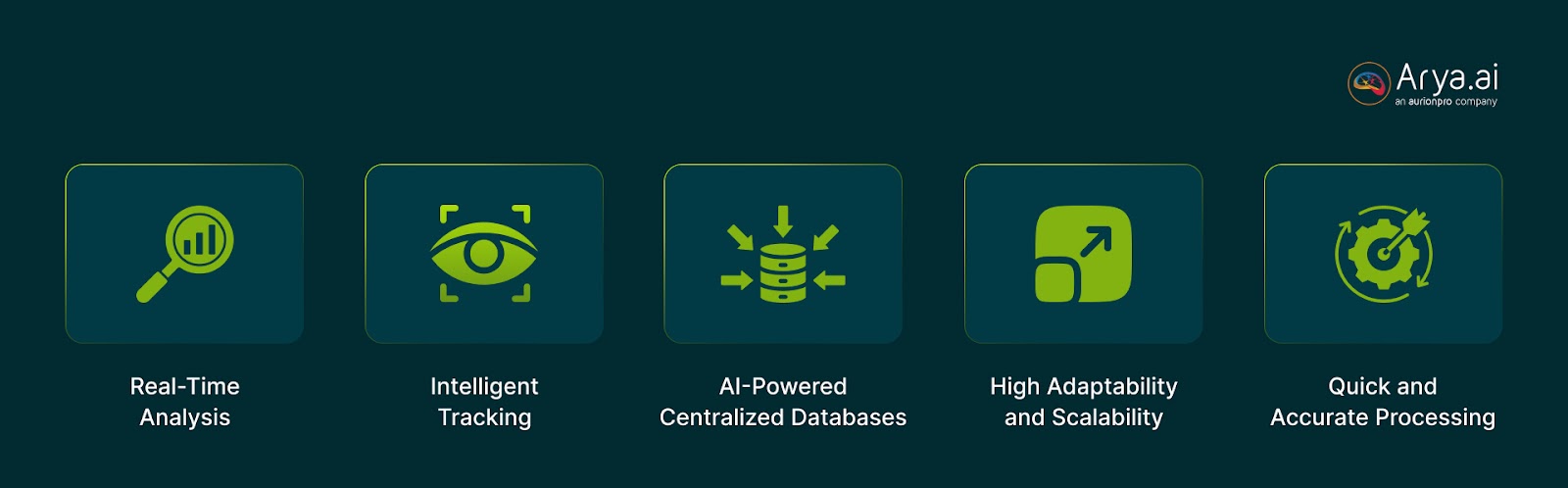
Here’s a detailed breakdown of how to detect mule accounts using AI:
- Real-time analysis: AI’s ability to process and analyze large volumes of data in real-time makes it ideal for spotting fraudulent patterns that traditional rule-based systems miss. AI can continuously monitor account activities, swiftly flagging anomalies such as unusual transaction frequencies or mismatched data points.
- Intelligent tracking: By integrating data from multiple sources (such as transaction logs, customer profiles, and behavioral analytics), AI creates a comprehensive view of account behavior. This makes it easier to pinpoint suspicious activity.
- AI-powered centralized databases: A centralized database is a unified system that collects, stores, and shares fraud-related information across multiple financial institutions. This improves coordination and detection efforts between banks. For example, if a fraudster uses multiple banks for mule account activity, a centralized database allows banks to cross-reference customer profiles, flagged transactions, and risk scores in real-time.
- High adaptability and scalability: AI systems are designed to learn from new data and adapt to evolving fraud tactics. This ensures that detection methods remain effective as fraudsters change their strategies.
- Quick and accurate processing: Advanced machine learning models process high volumes of transactions with high accuracy, This results in a significant reduction in the lag time between fraudulent activity and detection.
In short, AI transforms mule account detection from a reactive, manual process into a proactive, automated system that adapts to evolving fraud tactics. However, while Al offers significant benefits in fraud detection, banks must balance innovation with caution.
How Arya AI Combats Mule Accounts: Building Resilient Banking Systems
Arya AI leverages artificial intelligence to detect and dismantle mule account networks before they can do damage. By integrating deep learning, intelligent tracking, and real-time anomaly detection, Arya AI keeps banks protected from account-related frauds.
Through its intelligent document processing, Arya AI streamlines document verification, ensuring that every piece of financial data is accurately captured, analyzed, and authenticated.
Arya AI’s algorithms flag inconsistencies that human reviewers might miss. By analyzing behavioral patterns and transaction histories, Arya AI detects unusual account activity, distinguishing between genuine transactions and those designed to circumvent fraud detection systems.
Beyond fraud detection, Arya AI consolidates fraud-related insights across multiple channels and offers a unified monitoring system that strengthens anti-money laundering (AML) and Know Your Customer (KYC) protocols. Using Arya AI, financial institutions can mitigate risk with greater accuracy, reduce operational losses, and enhance regulatory compliance without burdening internal teams with manual investigations.
Conclusion
As fraud tactics grow more sophisticated, banks can no longer rely on outdated rule-based systems and manual reviews. AI-powered fraud detection is the future, enabling banks to track suspicious patterns in real-time and identify high-risk accounts before fraud occurs.
Solutions like Arya AI are leading the way, integrating advanced document verification, anomaly detection, and intelligent monitoring to provide a holistic approach to fraud prevention. By embracing AI, financial institutions can not only protect themselves from escalating fraud risks but also build stronger, more resilient banking ecosystems.
The fight against mule accounts is no longer optional, it’s necessary. The question isn’t whether banks should adopt AI for fraud detection, but how soon they can implement these solutions to stay ahead of financial criminals.