
What is Transaction Monitoring in AML?
Transaction Monitoring in AML is the process of continuously tracking financial transactions of customers to detect suspicious or illegal activities, like money laundering. A transaction monitoring system (TMS) helps a financial institution oversee and protect the quick transaction capabilities your customers expect—be it transferring, depositing and withdrawing money.
Importance of Transaction Monitoring in AML
Transaction monitoring ensures that the customers you deal with are trusted parties and are conducting transactions as per their profile. A Suspicious Transaction Report (STR) is filed if the system finds an unusual transaction.
Unusual transactions can be anything from frequent transfers, large deposits, high-value transactions or transfers to high-risk countries. Without transaction monitoring, organizations find it daunting to prevent financial crimes and comply with AML regulations.

Transaction Monitoring in AML: Rundown of the Process
The process of Transaction monitoring in AML includes analyzing patterns, deviations, and anomalies in customer behavior. It’s also about identifying risks over time through an ongoing review process. Here’s how it works:
1. Establishing Customer Risk Profile & Data Collection
Transaction monitoring starts with understanding a customer’s risk profile. This includes gathering KYC data—like occupation, country of origin, and source of funds—and continuously updating it with transaction history. Transaction data like the amount, date, time, sender and receiver numbers, and geographical location of transactions, are also collected.
Then, instead of treating all customers equally, monitoring intensity is based on individual risk levels. The more high-risk a customer’s profile, the more attention their transactions receive.
2. Creating and Implementing Monitoring Rules
Specific rules are set up within the TMS to detect unusual activities. Regulatory requirements, industry best practices and your institution’s risk appetite fall under the scenarios. Monitoring rules detect transactions that are significantly outside a customer’s normal behavior.
Some of these include transactions significantly larger or smaller than a customer’s usual activity, multiple transactions in rapid succession or showing a pattern that suggests trying to avoid the reporting threshold of the TMS, transactions from unusual or high-risk jurisdictions and known money laundering techniques.
3. Real-Time and Batch Monitoring
Transaction monitoring can occur in real-time or in batches. Transactions, when analyzed as they occur, allow immediate detection of potential risks, preventing threats like sanctions violations before they become an issue. This is real-time monitoring.
Batch monitoring looks for trends over time, where transactions are analyzed often on a daily or weekly basis. It should be used in conjunction with real-time monitoring as it provides a broader view of potential financial crime, that might not be apparent in individual transactions.
4. Alert Generation & Prioritization
When suspicious activity is detected, the TMS generates an alert. These alerts are prioritized based on risk factors, with higher-risk transactions getting immediate attention. The customer risk profile, transaction amount, or the gravity of the violated rule, all fall under these factors.
For example, a large cash transfer from a high-risk country may trigger a faster review than a minor irregularity, like a low-risk customer making a higher-than-usual transaction.
5. Manual Review & Case Management
Compliance analysts then investigate these alerts. They gather additional data, review the customer’s profile, and decide if the transaction warrants further action. If necessary, a Suspicious Activity Report (SAR) is filed to notify authorities of potential money laundering. Institutions must also maintain detailed records of these investigations and decisions taken, for a clear trial during compliance audits.
6. Continuous Optimization of Transaction Monitoring Systems
Transaction monitoring isn’t static. Rules are constantly updated, thresholds are adjusted, and new data sources are integrated. The goal is to refine the process, reducing false positives while keeping up with evolving threats.
Common Problems in the Present Status Quo of Transaction Monitoring
Traditional transaction monitoring in AML suffers from the following problems that reduce the efficiency of the entire AML framework.
1. High False Positive Rate
Around 90% of alerts generated by transaction monitoring systems are false positives, which drains the time and resources of AML analysts.
2. Low STR to Alert Ratio
The number of STRs filed compared to the total number of alerts generated is very low, meaning—many actual cases of money laundering and terrorism financing are not being detected.
3. Time-Consuming Investigations
Investigating a single alert can take anywhere between 60 minutes to 24 hours and more, as it requires collecting a lot of information about the case in question.
4. Failure to Detect Complex AML Cases
Criminals are becoming increasingly sophisticated and are finding new ways to conduct money laundering and terrorism financing activities. Traditional rule-based transaction monitoring systems are often unable to detect these complex cases.
5. Lack of Proper Information for Decision Making
AML analysts may not have access to all the necessary information to make informed decisions about whether a transaction is suspicious or not.
Recommendations for Developing an Effective Transaction Monitoring System
To build a strong transaction monitoring system, you can include the following foundational elements in your organization.
1. Linkage with KYC
Transaction monitoring should be linked with KYC (Know Your Customer) and customer due diligence processes. The better the organization is at KYC, the more effective its transaction monitoring system will be.
2. Capability Maturity Model in Transaction Monitoring
Your organization can use the Capability Maturity Model (CMM) to assess the effectiveness of its transaction monitoring system and find areas for improvement. It guides your teams from basic, inconsistent processes to advanced, efficient systems.
Each stage of CMM (from Initial to Optimizing) adds structure, control, and improvements. Your organization progresses from manual, error-prone checks to data-driven, highly efficient transaction monitoring with lower false positives and better risk detection. Using CMM in AML also makes your organization’s compliance stronger, since the monitoring system aligns with its risk profile.
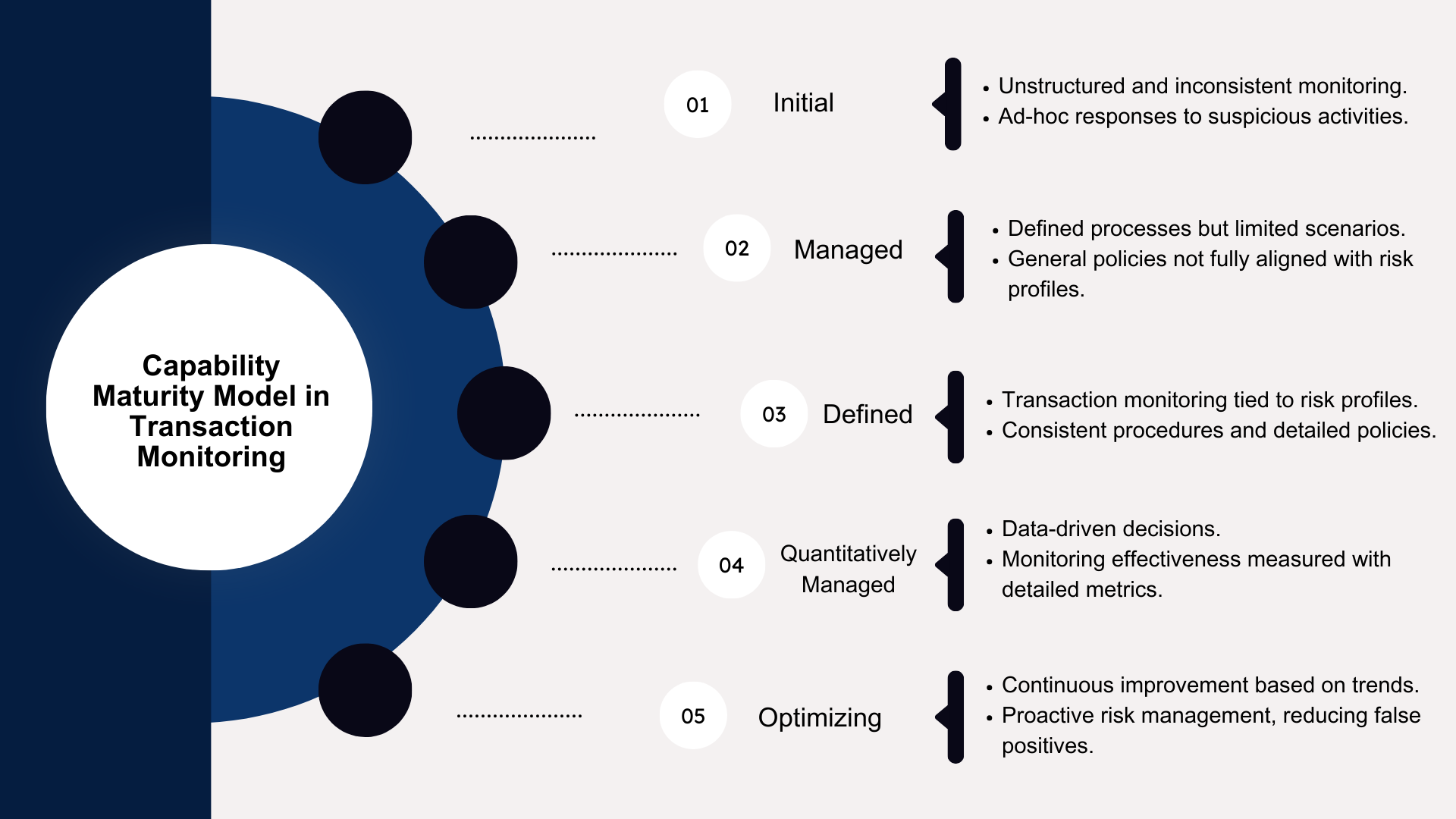
3. Risk-Based Approach:
Transaction monitoring should be based on the organization's risk profile. So, your resources should be allocated to monitoring high-risk customers and transactions.
4. 360-Degree View of Customer Information:
AML analysts need to have access to a 360-degree view of customer information, including all transactions performed by the customer.
With a strong foundation for transaction monitoring in AML, adding advanced technologies like AI can significantly enhance your TMS's ability to detect and prevent financial crime.
How AI is Revolutionizing Transaction Monitoring
AI can help organizations move away from traditional rule-based systems that flag only 10% of actual threats to advanced algorithms with the potential to analyze massive datasets and find even subtle patterns that point to financial crimes.
AI’s ability to detect unusual activities like multiple deposits or withdrawals in short periods improves efficiency and accuracy. It also reduces the burden of false positives on compliance teams by identifying transactions involving high-risk individuals or countries. Institutions have reported between 77% to 33% reduction in false positives.
AI also cuts down the time required for Suspicious Activity Reporting (SAR) with Natural language processing (NLP). Documenting and filing SAR reports requires significant manual efforts but NLP can automate the generation of SARs. AI-enhanced Customer Due Diligence (CDD) now uses real-time data sources. So, emerging patterns and anomalies, that may be new money laundering typologies, can also be detected. Verification accuracy gets a boost and your customers will benefit from much lower onboarding delays.
How Arya AI Helps with AML Transaction Monitoring
Arya's suite of products provides essential support to your financial institution in building a comprehensive AML framework.
KYC Extraction
Efficiently retrieves accurate data from Indian KYC documents, ensuring the verification process starts with precise customer information.
Document Fraud Detection
dentifies tampered documents, minimizing fraud and protecting your financial institution from onboarding fraudulent customers.
Bank Statement Analyser
Provides a clear financial profile of your customers by summarizing account details from bank statements, which also helps in monitoring financial behaviour.
Face Verification
Ensures that your customers are who they claim to be by comparing live images with stored photographs—a critical element in securing customer identification.
Deepfake Detection
Detects digitally altered images, audio, or video, providing another layer of protection in preventing identity fraud during onboarding.
Conclusion
Effective transaction monitoring is crucial for preventing financial crime and complying with regulations in the BFSI sector. AI helps financial institutions significantly improve the accuracy and efficiency of their AML frameworks. A well-integrated transaction monitoring software reduces false positives, speeds up investigations, and keeps up with evolving threats.
Arya AI's offerings complement these efforts, supporting KYC, fraud detection, and customer profiling. Staying prepared in AML transaction monitoring protects institutions from financial crime and compliance while developing customer trust.