.jpg)
Financial institutions have long embraced rule-based automation. Now, intelligent automation is on the horizon. Advancements in AI, and particularly generative ai, are making this possible.
Financial operations are data-intensive. It could range from accounts payable to complex forecasting, everything requires poring over large datasets. Financial institutions adopted OCR and RPA to ease this burden. The workflows that could be automated, however, remained straightforward. OCR in banking, for instance, enabled banks to store data from documents.
AI has brought about a paradigm shift, one that goes beyond task-based automation to an intelligent one. Let’s learn what it is and how it could fit into the broader automation landscape.
What is Autonomous Finance?
Autonomous Finance refers to processes that go beyond simple automation and are partly governed.
Systems under autonomous finance can operate with minimal human intervention and are driven by self-learning, adaptable software. These systems are typically powered by AI, ML, analytics, and sophisticated decision-making frameworks.
They monitor data in real time and interpret the influx of information through advanced algorithms. This then allows the systems to make proactive decisions, whether that is a simple task like invoice processing or complex processes like credit risk analysis.
The three elements that define autonomous finance are:
- Automation with minimal intervention
- Adaptive learning
- Predictive capabilities
Financial Automation: A Tiered Approach
To achieve autonomous systems, financial automation has to be approached as progressive automation. It begins with mapping where the organization stands today, where manual operations are the starting block.
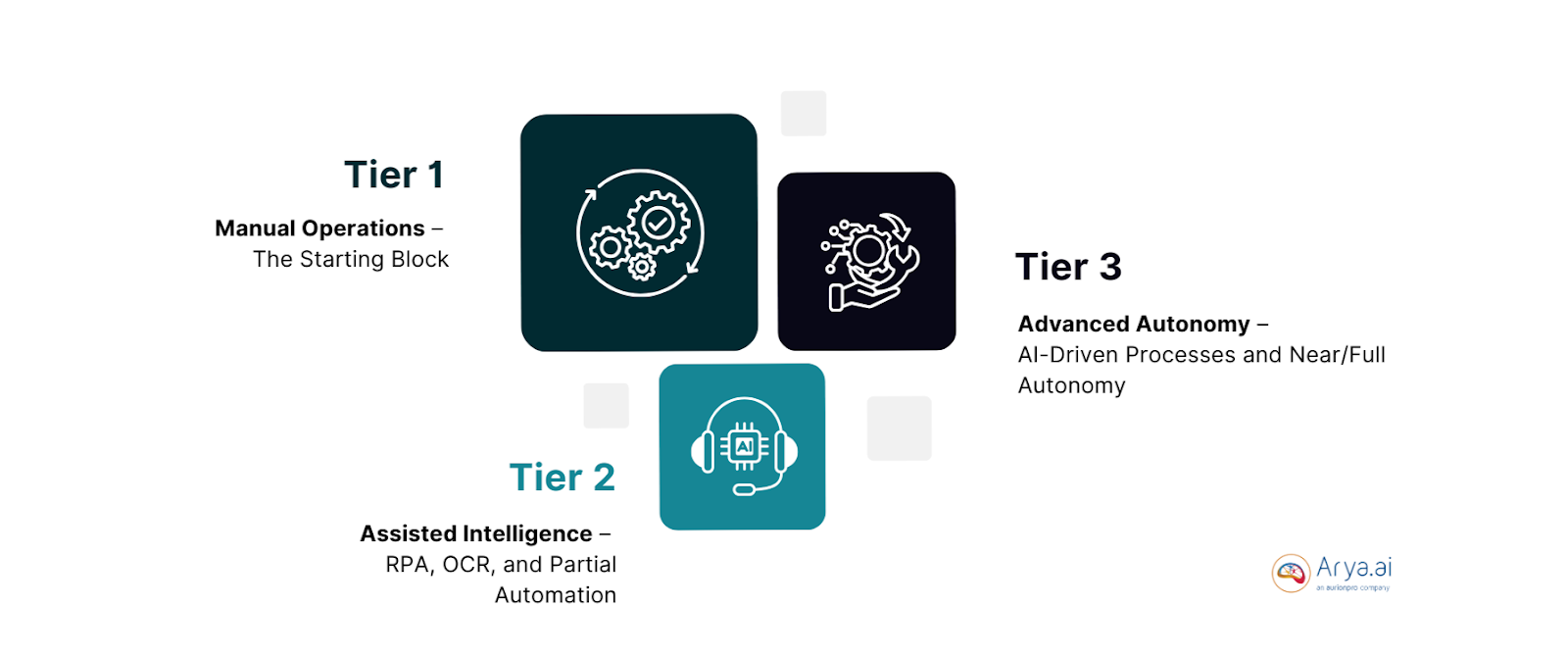
Tier 1: Manual Operations – The Starting Block
This is the stage where many organizations begin. It involves manual tasks, such as data entry, invoice processing, and reconciliations – all of which are handled by human operators. There is limited visibility because reporting is largely retrospective and manual.
Tier 2: Assisted Intelligence – RPA, OCR, and Partial Automation
At this stage, organizations begin to use specific technologies to assist human-led processes. Here, the following technologies play a major role:
- Optical Character Recognition (OCR): Reads and extracts data from paper-based or scanned documents to reduce manual data entry.
- Robotic Process Automation (RPA): Automates repetitive, rules-based tasks such as invoice entry or data migration.
This assisted intelligence frees teams to focus on tasks that require nuanced judgment or strategy. However, they do not offer adaptive or predictive capabilities; human intervention is still essential to guide the workflow and make complex decisions.
Tier 3: Advanced Autonomy – AI-Driven Processes and Near/Full Autonomy
This final tier is where Autonomous Finance comes into full view. Deep learning models, generative AI, and other advanced algorithms enable systems to learn continuously, predict outcomes, and make complex decisions with minimal human input. Real-time data monitoring and adaptive feedback loops let the technology self-correct, reducing errors and further optimizing the process.
Combined with robust predictive analytics, Tier 3 automation effectively transforms finance from a reactive back-office function into a proactive, strategic force—generating forward-looking insights, surfacing new revenue opportunities, and mitigating risks before they escalate.
However, reaching this stage requires not only high-quality data and advanced ML frameworks but also strong governance and comprehensive risk management to ensure compliance in highly regulated environments.
With the rise of generative AI, capable of handling large datasets, crafting detailed reports, or even simulating potential market scenarios, organizations at Tier 3 can explore entirely new frontiers of automation and insight. This level of autonomy sets the stage for a finance function that helps drive innovation and sustained growth.
Autonomous Finance vs AI Agents: Is There a Difference?
At a glance, Autonomous Finance and Agentic AI (or AI agents) may appear similar. Both leverage advanced technologies like machine learning, deep learning, or even generative AI to automate processes.
The key difference between autonomous finance and AI agents lies in domain specificity and governance requirements.
Autonomous Finance zeroes in on financial operations, emphasizing compliance, accuracy, and risk management. It’s designed to manage and optimize tasks ranging from day-to-day accounting to complex predictive analytics under strict regulatory guidelines.
Conversely, AI agents can be domain-agnostic, performing various tasks, like scheduling, customer support, or data retrieval, across different verticals.
Here’s a table demonstrating the difference between the two:
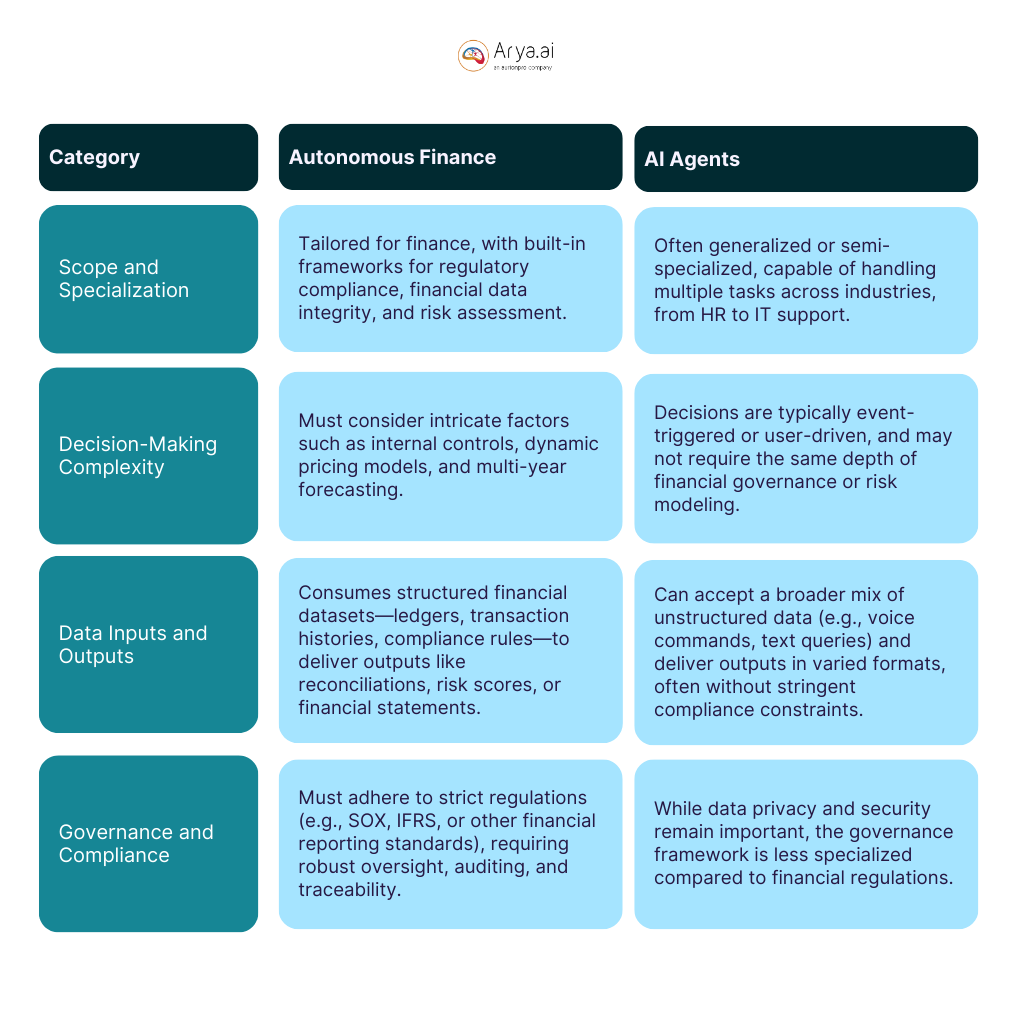
Roadmap for Adoption
Adopting Autonomous Finance requires a proper framework and roadmap.
1. Assess Current Processes: These are processes that require a lot of effort with minimal reward. So, identify high-volume, repetitive tasks for automation. If there is an existing technology driving these workflows, evaluate it.
2. Set Thresholds: Given the sensitive nature of financial data, we must define compliance requirements, risk thresholds, and escalation paths.
3. Implement Foundational Automation: We can begin with digitizing and automating repetitive tasks using basic technology, like OCR in banking, which can extract data from KYC documents.
4. Pilot AI-Driven Use Cases: This involves integrating sophisticated AI-driven solutions. We can first identify use cases, like an automated underwriting system. We can then continue to refine the models based on data and feedback.
5. Scale and Integrate: Once the integration is successful, scaling comes into the picture. As AI models learn and improve, they expand to new processes (e.g., enterprise risk management or procurement).
6. Monitor and Refine: Track process efficiency, cost savings, error rates, and user satisfaction. In addition, continuously update risk models and compliance parameters to adapt to evolving regulations and market conditions.
This roadmap ensures a measured transition from manual or partially automated tasks to truly autonomous finance processes.
Conclusion
Autonomous Finance represents the future of financial operations, pivoting away from rote, manual tasks toward intelligent, data-driven processes that proactively shape business outcomes.
This blog post covered the path of autonomous finance systems using a tiered approach, beginning with zero automation to advanced autonomy. The journey, however, requires proper planning, strong governance, and an appetite for continual improvement.
Arya.ai offers production-ready AI solutions to help organizations adopt advanced autonomy. Our solutions, like AI Cash Flow Forecasting and Intelligent Document Processing, allow enterprises to embrace intelligent automation.