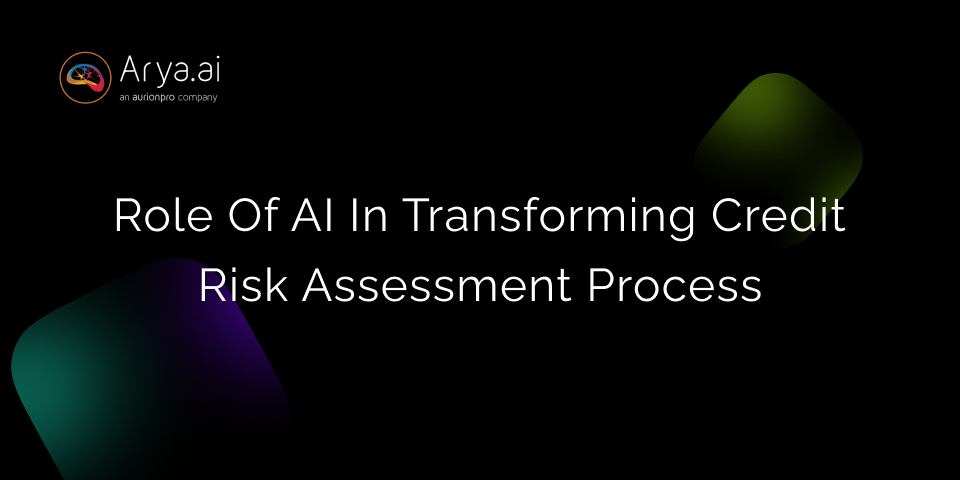
Credit risk assessment is a process that involves evaluating loan borrower’s creditworthiness by assessing their credit history, credit score, annual income, and more to determine their ability to repay the loan on time and the risks associated with it.
It’s one of the most critical processes for banks and financial institutions as it involves extensive strategic calculation, data analysis, and quantitative and qualitative analysis by the borrower.
When done manually, this process presents several challenges, including data entry errors, limited scalability, difficulty in data monitoring, accuracy risks, and time constraints. This is why banks and modern financial institutions are shifting towards automation and leveraging Artificial Intelligence solutions.
According to a report for global banking, McKinsey estimates that AI technologies could potentially deliver up to $1 trillion of additional value each year.

Thus, with more than half of operational tasks being automated and considering the vast data collection and analysis required in credit risk analysis, automated credit risk assessment is a must solution for banks.
In this article, we’ll explore AI's role in transforming credit risk assessment and its benefits for banks and financial institutions.
What is Credit Risk Assessment?
As discussed earlier, credit risk assessment or credit risk analysis analyzes a customer’s credit risk and likelihood of default on loan applications. This helps lenders like banks and financial institutions determine borrowers' creditworthiness and whether to reject or approve their loan applications.
This process involves analyzing several factors, such as the borrower’s financial history, credit score, debt-to-income ratio, income and employment, collateral, the purpose of the loan, and economic background and conditions.
Analyzing these factors makes it easier for banks and lenders to make better-informed decisions regarding the borrower’s loan application processing.
Traditional Approach to Credit Risk Assessment
Traditionally, the credit risk assessment consists of a manual review and evaluation of multiple financial factors with human intervention. It is often time-consuming and prone to human error, leaving banks vulnerable to banking fraud and financial losses.
However, since the manual traditional credit risk assessment process primarily relies on quantitative and qualitative analysis of the borrower’s financial information and other data manually, it comes with several challenges, including:
- Limited data utilization: Insufficient data analysis is one of the most critical limitations of manual risk assessment, as it primarily focuses on historical financial data and credit scores, overlooking other potentially relevant data sources, such as real-time transactions and social media activity, which could provide key insights into determining creditworthiness.
- Bias and subjectivity: Relying on human judgment to determine creditworthiness increases the risk of bias, subjectivity, and inconsistency, as lenders and decision-makers may have different perceptions, biases, experiences, and opinions, resulting in potential misjudgments and inconsistencies.
- Time-consuming: The traditional credit risk assessment process includes reviewing extensive documents, face-to-face interviews, manual credit scoring, financial analysis, and collateral evaluation—making it lengthy and highly time-consuming and limiting banks’ and lenders’ potential to make prompt decisions.
- Data quality and completeness: Since traditional credit risk assessment processes heavily rely on the completeness and accuracy of the provided credit reports and financial statements to make the right decisions, even slight inaccuracy and incomplete data can result in incorrect and inefficient risk assessments.
- Inflexibility: The reliance of traditional credit risk assessment approaches on rigid frameworks and standardized criteria makes them less flexible in evaluating or considering unconventional or emerging borrowers’ applications, like startups or freelance workers with less credit history or traditional income streams—resulting in higher rejection rates of potential credit=worthy applicants and missed opportunities for lenders.
- High costs: Manual credit risk assessment processes require significant resources and extensive expertise to analyze vast datasets and make accurate decisions, increasing operating costs for lenders and bankers.
- Compliance and regulatory burdens: Adhering to compliance and regulatory requirements with traditional credit risk assessment is highly complex and adds additional steps to the assessment process, burdening financial institutions and further slowing down the process.
Hence, integrating AI systems is essential to overcome these challenges and make the credit risk assessment approach more attainable, accurate, efficient, and adaptable.
How AI is Revolutionizing Credit Risk Assessment?
As we saw earlier, the traditional credit risk assessment approach has limitations. It overlooks certain factors required to analyze a borrower’s creditworthiness.
Hence, large organizations and financial institutions harness the power of technologies such as Artificial Intelligence (AI) and Machine Learning (ML) to streamline data analysis, ensure high accuracy, and make credible credit risk assessment decisions.
Further significant benefits of AI-powered credit risk assessment include:
- High accuracy and prediction capabilities
- Faster speed and efficiency
- Improved fraud detection
- Bias reduction
- Cost reduction
- Enhanced customer experience
According to a report, when a leading financial institution integrated an automated decision platform into their loan approval process in 2022, it resulted in a 50% deduction in decision-making time and a 20% overall increase in loan approvals.
This clearly indicates how AI enables dynamic risk assessment and real-time decision-making on loan approvals and accelerates credit underwriting to revolutionize and streamline credit risk assessment.
How AI-powered Credit Risk Assessment Works?
Here’s an idea of how AI-powered credit risk assessment works.

1. Data Collection and Integration
Besides traditional financial data sources, such as borrowers’ income, debt level, and credit scores, AI systems also collect data from alternative sources, such as utility payments, social media activities, audio and video data, and rental history, along with real-time transactional data, such as digital footprints and bank transactions.
This gathered data is integrated and aggregated into a unified system, offering a comprehensive view of a borrower’s financial stability and behavior.
2. Automated Global Credit Applications
An AI-powered credit risk assessment process allows banks and financial institutions to configure an online credit application system that facilitates customer onboarding and application form filling.
For instance, the system makes it easier for banks to configure and translate online application forms into any required language based on the global customer segment. The pre-configured and pre-filled credit application forms remove the need for customers to spend their valuable time filling out the forms, facilitating online credit application form filling.
3. Data Pre-processing
AI systems seamlessly handle data cleaning by cleaning raw data to remove inconsistencies and inaccuracies to ensure normalization and consistency. This includes correcting errors, handling missing values, and standardizing formats.
This step also includes the AI systems extracting relevant features from the raw data to be utilized in the predictive models, including calculating new metrics and aggregating and creating new data sensibly and meaningfully.
4. Automated Credit Scoring and Approval
Automated credit scoring is one of the key steps and benefits of an AI-powered credit risk assessment process. This process includes a system using advanced algorithms and predefined criteria to swiftly and efficiently evaluate an individual’s creditworthiness.
The system utilizes machine learning models like logistic regression, random forests, neural networks, and decision trees to analyze data based on historical factors to ensure credible decision-making, reliability, and accuracy.
5. Predictive Analysis
When trained, the AI risk assessment models can generate risk rankings and credit scores for new loan applicants based on their historical data and patterns. The generated scores reflect the borrowers’ default probability and other risk factors.
This real-time data processing and analysis enables immediate credit risk assessment, enhancing banks’ and lenders’ decision-making speed and capabilities.
6. Real-time Credit Risk Monitoring
AI systems enable real-time credit risk monitoring, allowing credit teams to monitor customer’s or borrowers’ profiles and track changes in their payment behavior in real-time.
Besides, they also receive real-time alerts and notifications in real-time regarding dips in credit scores and bankruptcy, allowing the teams to stay at the top of risks and manage credit risks effectively.
7. Continuous Learning and Improvement
Since AI models continuously learn from new outcomes and data, it improves their predictive accuracy over time and makes the credit risk assessment models adapt to changing economic conditions.
Besides, the lenders and credit team members regularly monitor and evaluate the performance of the AI models and metrics, such as precision, recall, accuracy, and more, to improve and assess the model’s performance.
Which Areas would Credit Assessment help businesses?
AI brings significant benefits to the credit risk management and analysis processes, including:
1. Credit Decisioning
Banks using the best AI models and algorithms offer much more accurate results and reports, enabling better credit forecasting and decisioning and reducing the turnaround time for credit approval.
Banks can improve lenders’ or borrowers’ risk determination, portfolio assessment, and credit approval by integrating essential tools, real-time analysis, structured and unstructured data, and automation.
Besides, AI-based algorithms also help banks determine a borrower’s maximum borrowing threshold, adjust pricing and credit offers, and offer competitive rates based on market changes.
2. Automated Data Collection and Monitoring
One of the significant benefits of AI-powered algorithms in credit risk assessment is automating data collection and processing vast amounts of data. AI systems efficiently gather and analyze borrowers' critical financial information, including their credit reports, financial statements, and transaction history.
Moreover, banks also use AI and ML-powered predictive analytics capabilities to predict potential risk accounts at an early stage and build a 360-degree monitoring perspective of a borrower’s portfolio. This allows banks to make the most of their collection systems and make accurate credit loan application decisions.
3. Improved Fraud Detection and Risk Mitigation
Another major benefit of credit risk assessment is detecting fraud more effectively. Real-time transaction data analysis identifies unusual patterns and anomalies to flag suspicious activities and prevent credit risks.
These advanced analytics enable financial institutions to detect potential credit risks in advance and prevent fraudulent transactions before they occur, minimizing the risk of financial losses.
Read now- Fraud Prevention Framework for Banks
4. Personalized Credit Offers
Offering personalized credit products and recommendations to customers is another major benefit of AI credit risk assessment.
While traditional assessment and lending systems offer one-size-fits-all solutions regarding credit recommendations, AI-driven credit risk analysis leverages ML algorithms to analyze vast data and gain insights into borrowers’ risk profiles, offering them tailored solutions that align with their requirements and creditworthiness.
5. Look for Early Warning Signals
Banks can analyze signs of potential fraud and risks through real-time data analysis and Natural Language Processing (NLP), preventing litigation and financial losses.
The ability to identify anomalies and suspicious patterns in transactions also helps banks prevent AI fraud, ensure a secure lending environment, and foster trust among borrowers and customers.
6. Dynamic Risk Assessment
Since AI and ML models continuously learn from new and changing data, they enable banks to assess risk dynamically through the borrowers’ updated risk profiles.
For instance, ML analyzes the borrowers’ economic changes, repayment patterns, and emerging risk factors, allowing banks and financial institutions to promptly adjust their decisions and risk assessment strategies, optimize capital allocation, and minimize losses.
7. Enhanced Customer Experience
Quicker credit decisions lead to improved customer experience as borrowers get timely responses to their credit applications from lenders and bankers.
Moreover, AI systems also offer tailored recommendations and personalized credit offerings to borrowers’ loan terms by analyzing their risk profiles, credit history, and data such as spending behavior and patterns to recommend solutions that meet their unique needs and preferences.
This significantly improves customer satisfaction and loyalty, which is highly beneficial for banks and financial institutions.
8. Bias Reduction
Properly trained AI models and credit risk assessment solutions lead to objective decision-making, reduce biases, and promote fairness for all customers and borrowers.
By leveraging advanced algorithms, continuous learning, analyzing and monitoring diverse data sources, and objective data processing, AI-powered solutions ensure an inclusive and fairer credit risk evaluation process.
The Challenges to Implement AI-Powered Credit Risk Assessment
While implementing AI-powered credit risk assessment provides significant benefits concerning cost reduction, accuracy, and high speed, it also comes with certain challenges and limitations.
Some of the challenges are listed below:
1. Data Quality and Availability
Access to quality data is a prominent barrier banks face when it comes to incorporating and adopting AI technologies in organizations—smaller banks are more likely to struggle than larger banks (approx. 25% to 28%).
AI models require large volumes of high-quality data for data analysis and to make accurate decisions.
However, financial institutions and banks may find it challenging to assess high-volume data, obtain quality data, and efficiently integrate the data stored in silos and dispersed systems—leading to inaccurate and incorrect credit risk assessment.
2. Bias and Ethical Concerns
AI models can inadvertently inherit and perpetuate biases in the historical data, which may contain algorithmic bias, leading to unfair treatment and potential discrimination towards certain groups in the credit risk assessment process.
3. Regulatory Compliance
Financial institutions and banks need to comply with changing and continuously evolving compliance standards and regulations, which can be challenging at first.
These models need to pass rigorous regulatory standards, which require them to be fair, transparent, and explainable.
4. Data Privacy and Confidentiality
A survey suggests that 80% of survey respondents feel protective about their financial/banking data and feel concerned about data breaches.
AI credit risk assessment requires access to confidential customer information, including their debt history, annual income, and payment patterns and behavior.
Hence, data privacy becomes critical to avoid breaches, misuse, and unauthorized access, which can otherwise lead to reputational and legal damages.
5. Lack of skills and expertise
22% of baking firms said that lack of talent and internal skills is a top barrier when it comes to incorporating AI technologies.
A shortage of skilled experts and professionals who understand AI technology and financial risk management is a significant challenge faced by financial institutions, especially small-sale banks.
Hence, continuous training and awareness of the staff regarding the benefits of AI, the latest AI advancements, and regulatory changes are essential to keeping them updated and on top of their game.
AI Credit Risk Assessment with Arya AI
Integrating credible and high-quality AI solutions that facilitate AI implementation to streamline banking operations and credit risk assessment is essential. Arya AI is at the forefront of this transformation, offering advanced solutions to enhance the precision and speed of your credit risk evaluations.
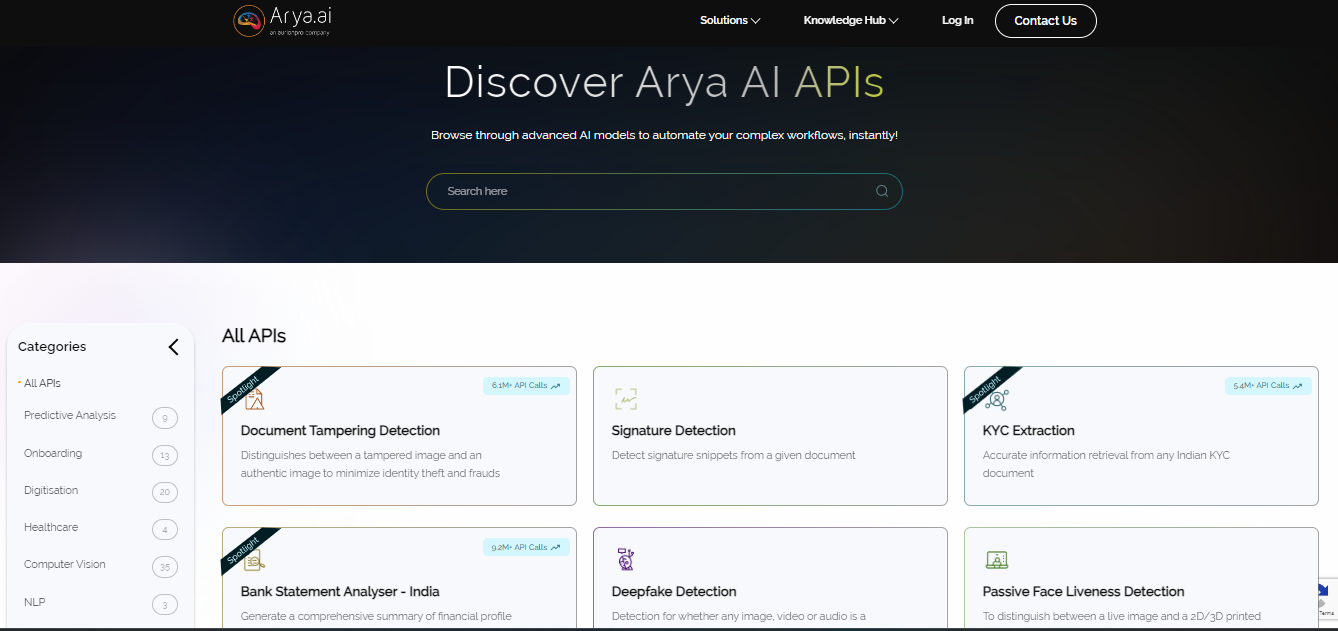
Our suite of APIs is designed to streamline and automate various aspects of credit assessment, ensuring you make well-informed lending decisions with confidence. You can directly integrate these AI APIs into your workflows and quickly create distinct processes according to your requirements!
- Bank Statement Analyser: Our Bank Statement Analyser API simplifies the process of reviewing and interpreting bank statements. By leveraging advanced AI algorithms, it quickly identifies patterns, assesses income stability, and detects any anomalies. This tool saves valuable time and ensures a higher degree of accuracy in evaluating an applicant's financial health.
- Document Tampering Detection: Security is paramount in credit risk assessment. The Document Tampering Detection API ensures the authenticity of submitted documents. Using sophisticated AI techniques, it identifies signs of tampering or forgery, protecting your business from fraudulent activities and ensuring the integrity of your credit assessment process.
- KYC Verification: Know Your Customer (KYC) compliance is essential for financial institutions. Arya AI's KYC Verification API automates the verification process, validating customer identities quickly and accurately. This ensures regulatory compliance and enhances the customer onboarding experience by reducing delays and manual intervention.
And many more APIs that help with credit risk assessment. Explore the APIs and try them for free with your own data on our platform- Arya AI.
Conclusion
AI may have its implementation and integration challenges, but when done right with the right talent, AI can transform and revolutionize the credit risk assessment process by enabling faster speed and high accuracy and mitigating fraud and data breach risks.
With personalized credit offers and recommendations, bias and fairness, data privacy, and enhanced customer experience, AI credit risk assessment helps banks stay ahead of the competition, reduce costs, and make better-informed decisions—essential to maintaining their reputation and legal liabilities.
Adopting Arya AI’s API is a good first step to ensuring responsible, effective, and accurate credit risk analysis and assessment. Contact us if you wish to join the AI bandwagon for your financial institution.