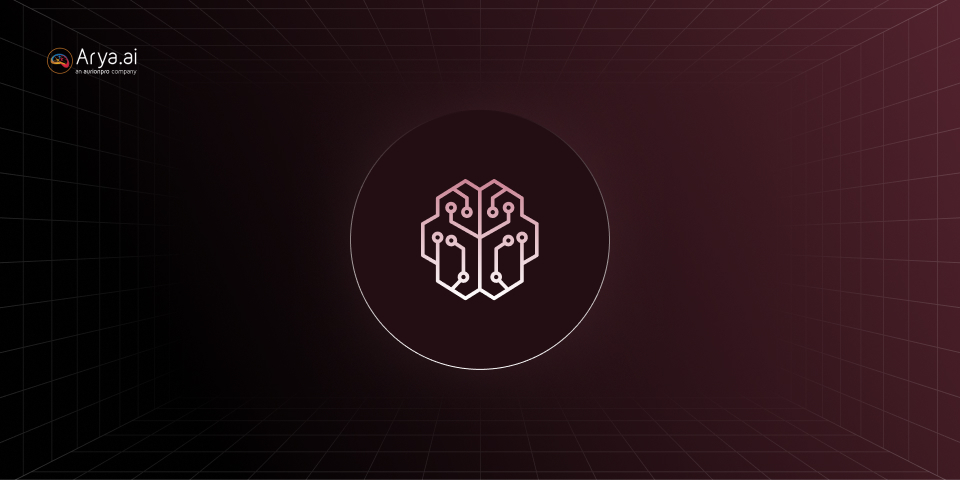
The rise of Large Language Models has done wonders for the financial industry. There are a number of things these models are capable of doing, and one such thing is financial analysis. These AI-powered models can analyze vast amounts of financial data, optimize risk assessments, improve fraud detection, and automate financial reporting.
From automated customer support to stock market predictions, financial LLMs are helping institutions increase efficiency, enhance security, and drive smarter decision-making.
What are Large Language Models (LLMs) in Finance?
Large Language Models (LLMs) are AI-powered deep learning models trained on massive datasets to process and generate human-like text. They use natural language processing (NLP) to interpret financial documents, market trends, and sentiment analysis in real time.
Key LLM Features in Finance:
1. Financial Sentiment Analysis
Analyzes sentiment in news, reports, and opinions to understand market trends, investor confidence, and sentiment-driven investment opportunities, enabling informed financial decisions and strategic planning.
2. Risk Assessment and Fraud Detection
Identifies anomalies in transactions or investments, leveraging AI to detect fraud and assess risks, improving security and compliance in financial operations while safeguarding stakeholders.
3. Automated Financial Reporting
Generates concise summaries of earnings, stock performance, and investments, reducing manual effort and ensuring consistent, real-time, and accurate financial communication across stakeholders.
4. Chatbots and Virtual Assistants
AI-driven chatbots provide instant, personalized financial advice, answer customer queries, and streamline service interactions, enhancing customer satisfaction and operational efficiency for financial institutions.
5. Predictive Analytics
Forecasts market trends, stock movements, and economic changes using historical and real-time data, empowering finance professionals to make proactive, data-backed investment and business decisions.
Top Financial Large Language Models (LLMs)
1. FinGPT
An open-source financial LLM designed for tasks like sentiment analysis and market forecasting. FinGPT can be fine-tuned swiftly to incorporate new data, making it adaptable to the dynamic nature of financial markets.
2. FinRobot
An AI agent platform that integrates various AI technologies for financial applications, extending beyond language models. It offers tools for market forecasting, document analysis, and trading strategies.
3. BloombergGPT
A 50-billion parameter LLM trained on a vast range of financial data, supporting diverse natural language processing tasks within the financial industry. It assists in improving tasks such as sentiment analysis, named entity recognition, news classification, and question answering.\
4. InvestLM
A financial domain LLM fine-tuned on LLaMA-65B, using a curated instruction dataset related to financial investment. It demonstrates strong capabilities in understanding financial text and provides helpful responses to investment-related questions.
5. FinLlama
Based on the Llama 27B foundational model, FinLlama is fine-tuned for financial sentiment classification, aiding in algorithmic trading applications. It classifies sentiment valence and quantifies its strength, offering nuanced insights into financial news articles.
LLM Use Cases in Financial Services
1. Fraud Detection and Risk Management
LLMs help banks identify fraudulent transactions by analyzing customer behavior and transaction history. They can also detect anomalies in real-time, reducing financial risks.
2. Credit Risk Assessment
By evaluating customer financial history, LLMs improve loan approval processes by predicting creditworthiness with higher accuracy.
3. Algorithmic Trading and Investment Strategies
LLMs analyze stock market trends, news, and historical data to optimize investment decisions. AI-driven trading algorithms can predict market movements more accurately.
4. Financial Document Processing
Banks and fintech firms use LLMs to automate financial reports, earnings summaries, and compliance documents, saving time and resources.
5. AI Chatbots for Financial Advisory
Financial institutions integrate AI chatbots powered by LLMs to provide personalized investment advice, customer support, and real-time insights.
Challenges of LLMs in Finance
Despite their benefits, LLMs in finance face challenges that institutions must address:
1. Data Privacy and Security
Managing sensitive financial data necessitates robust encryption measures and adherence to regulations like GDPR and FINRA to ensure security and regulatory compliance.
2. Bias and Inaccuracy
LLMs trained on biased datasets risk producing skewed financial predictions, impacting reliability and decision-making, and requiring vigilant dataset curation and testing.
3. High Computational Costs
Developing custom financial LLMs demands extensive computational resources, increasing expenses and requiring scalable infrastructure for effective model training and deployment.
4. Regulatory Compliance
AI models in finance must strictly follow industry regulations to mitigate legal risks, maintain trust, and meet compliance standards.
Future of LLMs in Financial Services
The adoption of financial LLMs is growing rapidly, with more firms integrating AI for data-driven decision-making. As models like BloombergGPT, FinGPT, and FinBERT evolve, they will continue to enhance automation, reduce fraud, and optimize investment strategies.
What is Next?
- Increased adoption of AI-powered financial advisors
- More custom-trained LLMs for banks and fintech firms
- Stronger AI regulations to ensure transparency and security
- AI-driven personalized financial planning and risk management
Conclusion
LLMs are transforming financial analysis by automating complex processes, improving fraud detection, investment analysis, and customer service. Whether it is BloombergGPT for finance insights, FinGPT for algorithmic trading, or GPT-4 for chatbots, LLMs are helping financial institutions stay ahead in the AI-driven economy.
Organizations that integrate AI-driven LLM solutions will gain a competitive edge in financial services. By leveraging financial LLMs, businesses can enhance efficiency, optimize risk management, and improve customer experience.
Financial institutions can explore AI-powered LLMs to enhance their financial operations today.