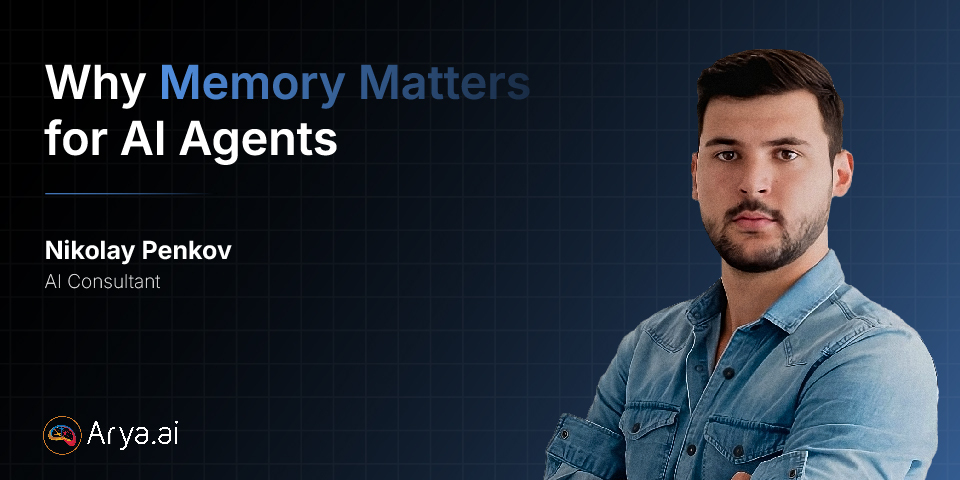
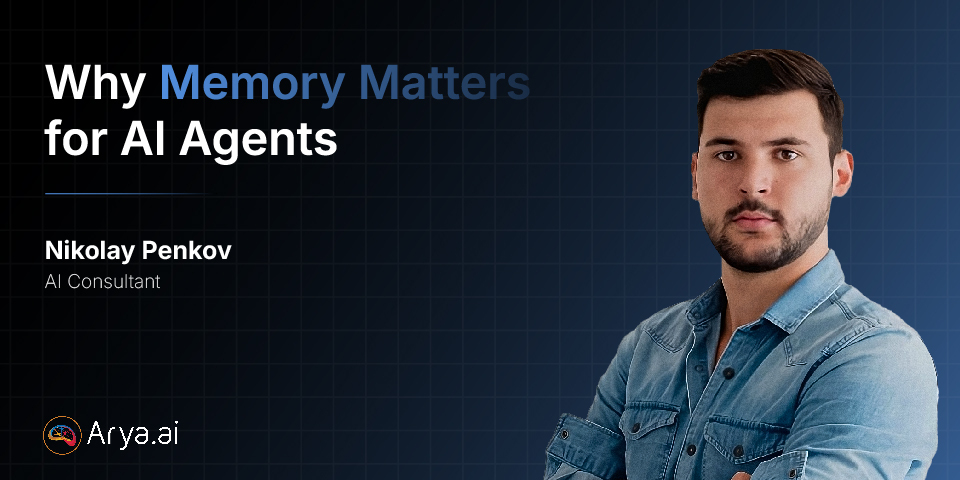
The blog post is a special part of the series we are doing with experts in AI, Development, Finance, and more. For this iteration, Nikolay Penkov, an AI Consultant, penned down his insights on why memory matters for AI agents and where it is headed.
Agentic AI is fast becoming one of the most prevalent trends. It takes actions autonomously, adapts in real-time, and solves problems in adversarial contexts. Traditional AI and then Generative AI showed great initial promise in addressing these. Agentic AI, however, transcends this beyond routine tasks.
Non-Agentic rule-based AI systems automate routine tasks, so their rigid adherence to predefined rules fundamentally limits them. This constraint hinders its ability to adapt, learn, and make independent decisions. Hence, they have proven inadequate in addressing the dynamic needs of modern enterprises.
Agentic AI improves enterprise operations by delivering end-to-end solutions that can autonomously achieve business objectives.
How AI Agents can perform the actions autonomously relies heavily on what they remember from information and interactions.
In this post, we explore:
- Why memory is central to creating intelligent, context-aware AI agents.
- The different types of memory (Procedural, Semantic, and Episodic).
- The importance of short-term and long-term recall.
- Strategies for deciding what AI should remember (and what it should forget).
Why Does Memory Matter in AI Agent Interactions?
Memory matters in AI agents for three main reasons:
- Context retention & dynamic interactions
- Predictive capabilities
- Personalization for a tailored experience
AI agents often handle multi-turn conversations, where past questions, user intent, and even subtle contextual clues are crucial for producing coherent answers. Without a mechanism for retaining context, agents risk providing irrelevant or repetitive responses.
Memory also plays a critical role in pattern recognition. In finance, for example, an AI system that can recall historical interest rate changes, transaction patterns, and user behaviors is better equipped to spot anomalies, predict market shifts, or personalize services.
From chatbots to recommendation engines, personalization is the name of the game. Agents who remember user preferences, past interactions, or browsing habits can tailor their responses, making every experience feel more intuitive and “human-like.”
What are the Types of Memory AI Agents Have?
.png)
There are three kinds of memory in an Agentic AI system:
1. Procedural Memory
Procedural memory encompasses learned behaviors and patterns, often through reinforcement learning or repeated training on tasks.
Application:
Think of a chatbot that consistently applies steps to verify user identity or a credit-scoring model that follows learned procedures to analyze risk.
2. Semantic Memory
Semantic memory stores general knowledge and facts—like domain-specific terminology or rules. This can be your system’s knowledge base, filled with definitions, historical data, or relevant industry guidelines.
Application:
A finance-focused AI might retain up-to-date compliance regulations, interest-rate trends, or fundamental economic concepts.
3. Episodic Memory
Episodic memory in AI involves remembering specific events or experiences, akin to recalling the who, what, where, when, and why.
Application:
Storing detailed chat histories or user interactions to maintain continuity in extended conversations or track unusual sequences of user behaviors.
What is Short-Term vs. Long-Term Memory?
Let’s explain this using a table:

What Should AI Agents Remember?
AI models should provide a balance between functionality, ethics, and compliance.
From a technical standpoint, all data is valuable for the best user experience. There is a famous proverb in the AI field emphasizing the importance of all bits of data, which goes like, 'There’s no data like more data.’
However, on the ethical side, AI solutions should safeguard personal information, avoid bias, and prevent echo chambers from past user prompts. This makes the memory aspect of agentic AI a complex topic, requiring robust regulations and security.
An important technical consideration for the performance of AI Agents will be the quantity of data processed when prompted.
There are two scenarios:
- Scenario 1: The AI agent already has internal knowledge about the prompted topic
- Scenario 2: The AI agent must access an external repository to extract knowledge about the prompted topic
When using external data, AI agents will be required to work with a limited amount of it so that the user experience (e.g., response latency) does not suffer. This limitation will decrease the details in the understanding of the prompted topic if the agent does not contain the information internally.
AI companies will let users create custom-tailored agents by embedding essential information with fine-tuning. This will equip the agents with the specific knowledge needed to perform tasks efficiently. However, this will also raise concerns about how the “learned” data is contained and secured.
The aforementioned memory blocks of an AI system are called “vector databases.” They store the external data in “embeddings” - a numerical form, capturing the semantic meaning and allowing for an efficient and scalable way of finding the most relevant information.
Storing user interaction data, even in vectorized form, can raise obligations such as the "right to be forgotten" when handling private user information. However, publicly available external sources, like internet websites, are commonly used as the go-to data sources—provided they do not explicitly prohibit use by AI systems.
Nevertheless, here is a list of specific information that should not be accessible to or retained by the AI agent unless explicitly requested:
- Financial details
- Medical records
- Passwords
- Credit card numbers
- Any other security-related credentials
- Anything shared incidentally
Where is it Heading?
We are going to see companies prioritize compliance and user protection while trying to maximize the usefulness of their AI products by utilizing all rightfully accessible data.
Safeguarding is already happening in different ways:
1. Memory Isolation and Encryption
User data should remain accessible only by the specific user. Personal data will be encrypted at rest to ensure that it’s stored securely.
2. Data Anonymization
Sensitive data should be completely removed from the AI agent memory to prevent harmful acts or misuse in high-risk systems.
3. Role-Based Access Control
Specific data should be accessible to an AI agent based on the prompting user's role, ensuring that the AI system provides information only to the appropriate user and within their authorization level.
Compliance and Regulation
Different business sectors are legally required to handle data with higher transparency and security. This includes adhering to industry-specific regulations, implementing robust data protection measures, and ensuring that all data processing practices comply with local and international laws.
AI is here to stay, and despite the various compliance rules and regulations, we will continue to see advancements in its performance and capabilities. While AI models are already sophisticated enough to deliver significant value, challenges such as model hallucinations and lack of specific knowledge hindered previously large-scale business adoption. However, the recent introduction of AI agents with enhanced memory and reasoning capabilities is addressing these issues and resolving these obstacles.
AI agents will not replace the human factor, but instead, they will empower people to leverage their time more effectively by providing quick and scalable expert-level insights on a large plethora of topics, for a fraction of the cost.
A Special Question
Q. In the financial domain, how do you envision memory playing a role in identifying and adapting to new risks or compliance regulations?
As AI grows more sophisticated and deeply integrated into enterprise workflows, memory emerges as a vital feature that powers coherence, adaptability, and intelligence.
Whether recalling procedural steps, factual data, or past interactions, well-designed memory systems enable AI agents to:
- Maintain context and provide consistent interactions.
- Predict trends by leveraging historical data.
- Adapt to specific domain needs, such as finance, healthcare, or customer service.
- Respect ethical and regulatory boundaries while storing and recalling information.
We are entering a new era of financial agility as AI agents will transform risk and compliance from topics that react to problems into valuable strategic tools. The benefits of memory in AI agents will allow for faster adaptation to black swan events, lower operation costs through automation, and stronger customer relations via transparency and explainability. The adoption of agentic AI will impact organizations positively and strengthen their operations and agility.