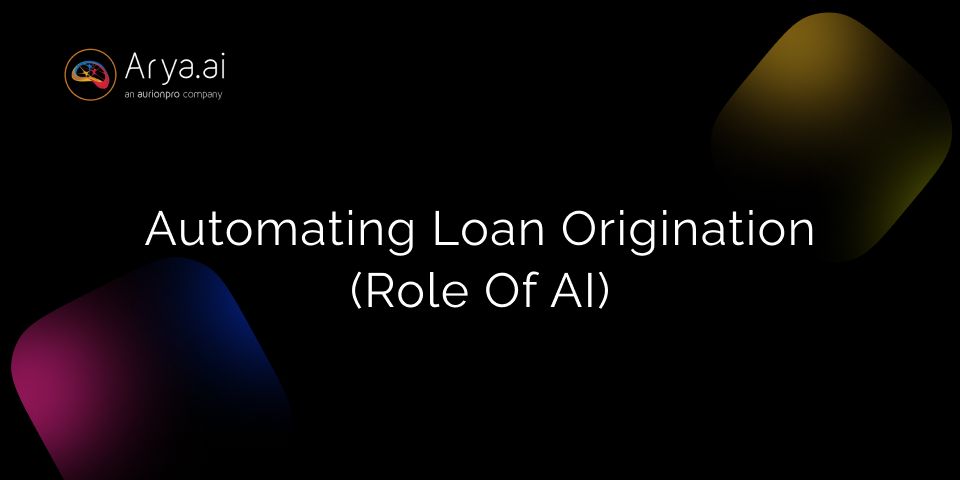
As technology continues transforming the finance sector, financial institutions are turning to Artificial Intelligence (AI) to streamline complex processes, and loan origination is no exception. Traditionally, loan origination has been labor-intensive and costly, involving multiple steps from approval application to meticulous verification. Each stage demands time, resources, and meticulous verification. Yet, as AI advances, this landscape is changing.
The numbers tell a compelling story. UK banks have been fully automating the loan underwriting process up to US$100,000. With the use of AI, there's an expectation of a 27% productivity increase across investment banks and 27%–35% front office employee productivity by 2026. A report suggests that by 2030, traditional financial institutions can save up to $31 billion of their underwriting and collection system costs by implementing AI technologies.
The COVID-19 pandemic intensified several current underwriting concerns: volume, speed, and risk. Robotic process automation, ML models, and different data sources can accelerate the loan underwriting process and improve risk evaluation. This process can be accelerated by automating document scanning and manual processes involved in gathering relevant data. ML models can run on data gathered from multiple sources (e.g., social media posts and third-party data). They can accurately assess borrowers' risk and quickly make loan decisions.
With the rapid evolution of AI, the transformation of loan origination from a manual, paper-heavy process to a seamless, tech-driven one is inevitable. In this article, we'll explore how AI is reshaping loan origination, the key technologies driving this transformation, and its real-world impact on banks and borrowers alike.
Current State of Loan Origination

Loan origination has traditionally been a multi-step, labor-intensive process, often involving a high degree of manual intervention.
1. Application and Initial Data Collection
- Current Process: Borrowers apply—often through physical forms or digital portals—which include personal, financial, and employment details.
- Challenges: Traditional systems rely on manual data entry, which is time-consuming and prone to error. Physical documents require secure handling and storage, adding to logistical and operational costs. The volume of data makes it challenging to review applications quickly, causing delays and often impacting customer satisfaction.
2. Credit Assessment and Scoring
- Current Process: Credit scoring in traditional loan origination relies heavily on credit bureau data to evaluate a borrower's risk level. Many institutions have fixed scoring criteria, which may not fully capture a borrower's true risk profile, especially for individuals without a lengthy credit history.
- Challenges: Relying solely on traditional credit scores can limit credit access and overlook non-traditional indicators that may provide a more nuanced picture of creditworthiness.
3. Document Verification and Compliance Checks
- Current Process: Verifying documents is essential for accurate underwriting. Compliance checks are required to ensure all applications meet regulatory standards and anti-money laundering (AML) requirements.
- Challenges: Manual verification involves significant time and resources, especially when processing documents with varying formats or languages. Errors or oversights during verification can lead to regulatory fines.
4. Underwriting and Decision-Making
- Current Process: Traditional underwriting relies on standardized guidelines, and loan officers typically make final decisions based on pre-set scoring models.
- Challenges: Manual underwriting is time-intensive and subjective, resulting in inconsistent decisions. Underwriters may apply guidelines differently based on their judgment, introducing variability into the approval process.
5. Approval and Disbursement
- Current Process: Once a loan is approved, it moves to the disbursement phase, where funds are released to the borrower's account.
- Challenges: Manual processes can slow down disbursement. This final stage often requires coordination between departments to ensure all conditions are met, which can lead to bottlenecks.
How AI is Transforming Loan Origination

AI is transforming the loan origination process through automation solutions that streamline and optimize every stage. It reduces manual work and leverages machine learning and predictive analytics. Here's how AI is transforming each critical step in the loan origination process:
1. Automated Data Collection and Analysis
- Impact: AI-powered loan origination solutions automate data collection, aggregating data from multiple sources such as credit reports, bank statements, and transaction histories.
- How It Works: With Natural Language Processing (NLP) and Optical Character Recognition (OCR), AI can instantly digitize and interpret unstructured data, such as scanned documents and handwritten forms, reducing the need for manual data entry.
2. Enhanced Credit Scoring and Risk Assessment
- Impact: AI-based lending automation is shifting traditional credit assessment methods to a data-rich, predictive model that draws from conventional and alternative data sources.
- How It Works: Machine learning algorithms analyze multiple variables, including income, spending patterns, employment stability, and behavioral data, to predict a borrower's repayability.
3. Streamlined Document Verification
- Impact: Verification of documents is often a bottleneck in loan processing. AI-driven solutions automate this by cross-checking documents against trusted databases, reducing manual work, and ensuring compliance with regulatory standards.
- How It Works: This automated loan origination capability speeds up loan approvals by flagging real-time discrepancies, allowing loan officers to focus on cases requiring manual intervention.
4. Predictive Decision-Making in Underwriting
- Impact: Traditional underwriting relies on static scoring models, but AI-based underwriting allows lenders to apply dynamic, data-driven decision-making that reduces biases and improves loan performance.
- How It Works: AI algorithms evaluate an applicant's creditworthiness based on patterns and trends from historical loan data, allowing for a more nuanced risk assessment.
5. Faster and More Secure Approval and Disbursement
- Impact: Automated loan processing systems expedite approvals by automating compliance and risk checks to meet regulatory standards.
- How It Works: AI-driven loan origination solutions incorporate predictive analytics to ensure each application meets compliance and risk guidelines before final approval.
Benefits of AI in Loan Origination
1. Increased Efficiency and Faster Processing
- Accelerated Application Review
- End-to-End Automation
2. Enhanced Accuracy and Reduced Errors
- Minimized Manual Data Entry
- Improved Document Verification
3. Improved Risk Management and Credit Assessment
- Advanced Credit Scoring Models
- Predictive Risk Assessment
4. Enhanced Customer Experience
- Quicker Approval Decisions
- Personalized Lending Solutions
5. Cost Savings and Operational Efficiency
- Reduced Operational Costs
- Scalability
6. Enhanced Compliance and Fraud Detection
- Real-Time Compliance Checks
- Fraud Detection
7. Better Data Utilization and Insights
- Data-Driven Decision-Making
- Continuous Model Improvement
Challenges and Considerations in AI-Powered Loan Origination
1. Data Privacy and Security Concerns
- Challenge: AI systems require access to vast amounts of sensitive data to make accurate lending decisions. This necessity raises concerns about data privacy, security breaches, and compliance with data protection regulations like GDPR or CCPA.
- Consideration: Financial institutions need robust data encryption, secure storage solutions, and strict access controls to protect customer information.
2. Bias in AI Models
- Challenge: AI algorithms can unintentionally perpetuate or amplify existing biases in lending decisions, leading to discrimination against certain groups.
- Consideration: To address bias, institutions should regularly audit their algorithms, use diverse training data, and apply bias-mitigation techniques.
3. Regulatory Compliance and Accountability
- Challenge: As AI decisions may lack transparency, they can complicate compliance and accountability for regulatory requirements.
- Consideration: Financial institutions should implement AI models that are both explainable and auditable, allowing for clear documentation of decision-making processes.
4. Model Accuracy and Performance Risks
- Challenge: In dynamic financial environments, AI models may fail to account for unforeseen factors such as economic downturns, changes in consumer behavior, or new fraud tactics.
- Consideration: Using machine learning techniques that adapt to new data can help improve model performance and ensure it remains relevant to changing conditions.
5. High Initial Investment and Ongoing Costs
- Challenge: Deploying AI in loan origination requires substantial upfront investments in technology, skilled staff, and infrastructure.
- Consideration: Partnering with experienced vendors or adopting modular AI solutions can reduce initial investments and allow for a phased implementation approach.
6. Lack of Human Oversight and Customer Trust
- Challenge: A fully automated loan origination process may lead to concerns over impersonal service and a lack of empathy.
- Consideration: Incorporating human oversight at critical points, such as final loan approval or customer support, can provide a balanced approach to AI-driven processes.
The Future of AI in Loan Origination
1. Hyper-Personalization of Loan Products:
Leveraging AI and big data, financial institutions will offer increasingly personalized loan products. This hyper-personalization could involve flexible loan terms, dynamic interest rates based on real-time risk assessments, or customized repayment plans.
2. Real-Time, AI-Driven Decision-Making:
Real-time AI-driven decision-making will be at the core of future loan origination processes. Instant credit assessments will enable "one-click" loan applications where approvals are nearly instantaneous, eliminating long waiting periods and paperwork.
3. Enhanced Fraud Detection and Security Protocols:
AI in loan origination will increasingly focus on predictive fraud detection to identify potential fraud before it happens.
4. Integrating Alternative Data for Broader Accessibility:
The use of alternative data—such as social media activity, online spending behavior, and even mobile usage patterns—will provide a fuller picture of an applicant's financial habits, particularly for those with limited credit history.
5. Greater Use of Explainable AI (XAI):
Explainable AI (XAI) will become increasingly vital as regulators and borrowers demand transparency in AI-driven lending decisions.
Conclusion
AI's role in loan origination is not just transformative; it's redefining how financial institutions approach lending from the ground up. By automating complex, time-consuming processes, AI enhances speed, accuracy, and efficiency, allowing lenders to meet the expectations of modern consumers and businesses who demand faster, more flexible loan solutions.
The future of loan origination is clearly AI-powered. As lenders continue to adapt to evolving technology, they will be better positioned to offer hyper-personalized, secure, and compliant lending experiences that cater to the diverse needs of borrowers.