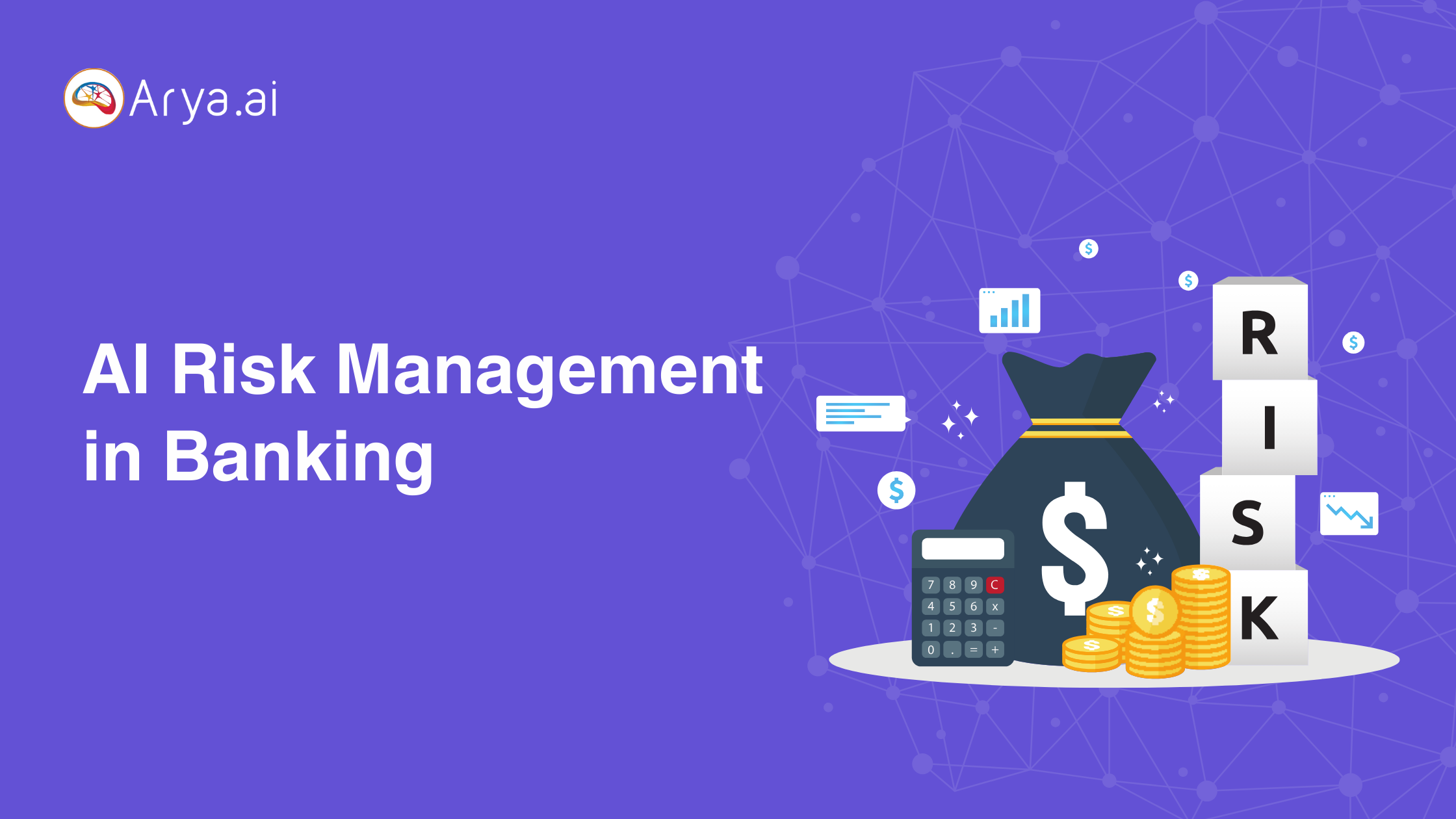
With banks and financial institutions heavily relying on large volumes of data, they are continuously leveraging Artificial Intelligence (AI) and Machine Learning (ML) technologies to speed up processes, reduce costs, and better serve their customers.
But the most critical aspect AI impacts and helps is risk management. Banks face several types of risks, including credit, transaction, compliance, liquidity, and reputational risks.
While the banking industry is at the forefront of adopting new tools and technologies to stay ahead of the curve, according to the PWC survey, 79% of banks and businesses report that keeping up with the accelerating digital transformation is one of the major risk management challenges.
Thus, having a robust AI risk management system that not only helps curb banking risks but also streamlines operations and better serves the customer is crucial.
In this article, we’ll see how AI helps banks and businesses transform risk management and its key benefits and applications in today’s world.
What is AI Risk Management?
AI risk management refers to using a suite of tools and Artificial Intelligence (AI)- based practices to protect banks and financial institutions from various risks, including fraud, credit card risks, operational risks, cybersecurity risks, and more. It involves identifying, assessing, measuring, and mitigating risks in the financial sector.
For instance, AI risk management uses AI algorithms for fraud detection, credit risk management, and compliance monitoring to help banks make better-informed decisions, improve risk management efficiency and accuracy, and automate risk management processes.
How is AI changing Risk Management?
Artificial Intelligence is significantly changing, or rather, transforming risk management by improving efficiency, boosting productivity, and reducing costs.
AI can quickly analyze large volumes of data without any human intervention to provide key insights regarding existing and emerging risks, making it easier for risk managers to make better decisions.
Before the advent of AI, traditional risk management processes relied heavily on manual operations and rule-based approaches. For instance, banks manually assess historical data based on predetermined rules to analyze and detect different risks.
The traditional risk management processes were highly time-consuming, inefficient, labor-intensive, and prone to human errors because of manual data collection, analysis, assessment, and decision-making.
AI in risk management helps banks and financial institutions overcome these issues, making the whole process more agile, accurate, secure, and efficient.
Importance of AI Risk Management in Banking
AI-driven risk management offers unparalleled advantages to banks and financial institutions in combatting risks and boosting their precision and accuracy.
The Indian Forensic Research suggests that the insurance sector loses up to ₹30,401 crores each year due to banking fraud risks, making the insurance companies lose around 8.5% of revenue.
Since AI-powered risk management processes use sophisticated tools and technologies to combat risks by better analyzing data and offering key insights, it helps banks identify potential risks and empower better decision-making.
AI’s data-driven insights and ability to automate complex risk management processes reduce the involvement of human resources, the possibility of errors, and the likelihood of unexpected setbacks, enabling risk managers to focus on more strategic and innovative tasks.
Thus, AI in risk management allows banks to anticipate and mitigate risks effectively by creating a secure, stable, and streamlined operational environment.
What are the key benefits of using AI in Banking risk management?
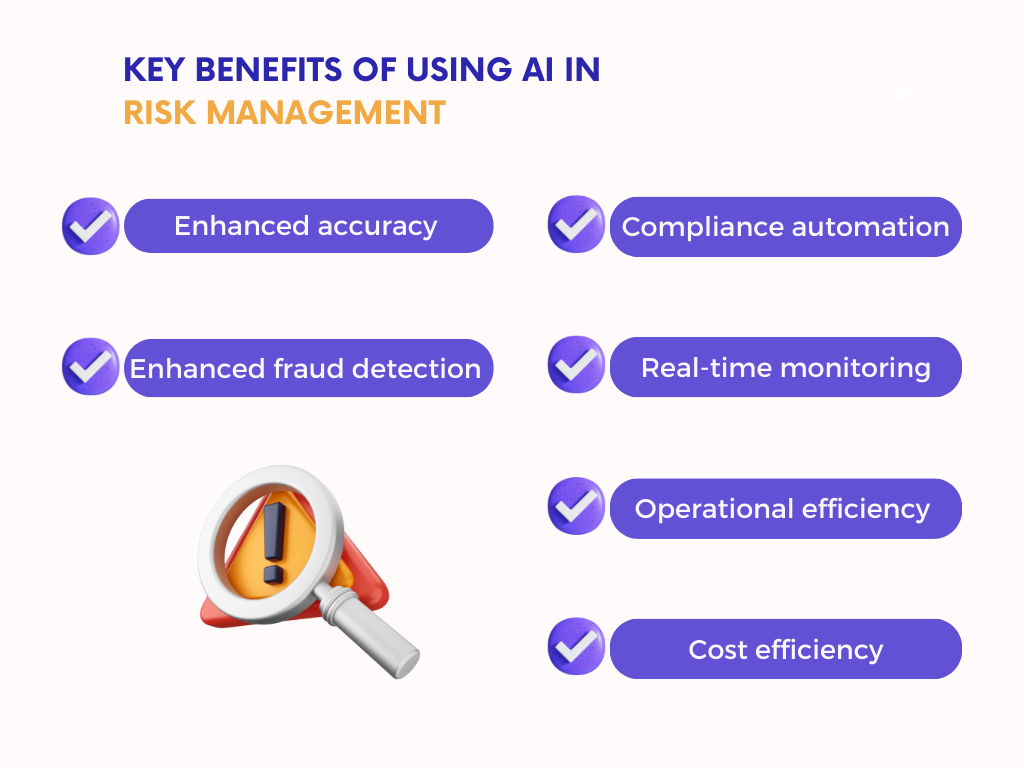
AI unlocks several benefits for risk management in the banking industry. Below are some of the key benefits.
1. Enhanced Accuracy
Artificial Intelligence algorithms excel at analyzing vast volumes of data and identifying patterns, behavior, and anomalies that a human risk manager could not apparently identify.
By leveraging advanced analytics, predictive analysis, behavioral analytics, and real-time monitoring, AI risk assessment systems can identify, assess, and mitigate risks more effectively and accurately than traditional models.
2. Enhanced Fraud Detection
AI facilitates fraud detection in risk management through advanced data analytics, real-time monitoring, and pattern recognition.
AI algorithms can easily identify outliers and unusual patterns in transaction data, flagging fraudulent activities and unusual behavior that deviate from normal transactional behavior.
Thus, by continuously analyzing a huge dataset, AI risk management helps banks and financial institutions stay ahead of the curve and prevent emerging fraud risks.
3. Compliance Automation
AI systems and algorithms help banks ensure compliance with standards and regulations by providing transparent and consistent risk management processes and ensuring adherence to regulatory requirements.
AI systems continuously monitor regulatory updates and changes by monitoring and analyzing official announcements and regulatory documents, to assess their impact on the banks or organizations, ensuring compliance with changing legal requirements.
Thus, by automating risk assessment, transaction monitoring, regulatory monitoring, policy enforcement, documentation, and data privacy compliance, AI risk management streamlines compliance processes, making it easier to mitigate compliance risks more effectively.
4. Real-time Monitoring
AI ensures real-time monitoring by monitoring data streams, including transactions, user behavior and activities, and market data, to identify and provide timely alerts about potential risks and deviations.
This real-time monitoring enables proactive risk management by preventing potential threats from hampering the banks’ or financial institutions’ operations.
5. Operational Efficiency
By automating manual processes, such as document analysis, data entry, data processing, report generation, and other mundane tasks, AI in risk management reduces the chances of human errors and frees up human resources, allowing them to focus on much more strategic tasks.
Thus, by automating repetitive tasks, AI enables faster data analysis, optimizes resource allocation, and streamlines decision-making, improving the banks’ overall operational efficiency and productivity.
6. Cost Efficiency
AI significantly reduces manual efforts and resources by automating analysis processes and repetitive tasks, helping banks reduce costs associated with risk management. ]Reducing costs enables banks and financial institutions to invest that revenue in more advanced tools and technologies that help them boost their accuracy, productivity, and efficiency to prevent risks, enabling long-term cost savings.
What are AI Risk Management Technologies for the Banking Industry?
Banks need to leverage advanced technologies to identify and mitigate several types of risks and ensure efficient risk management. Here are some of the critical technologies that banks use when implementing risk management.
1. Artificial Intelligence (AI)
Banks and financial institutions use Artificial Intelligence technologies to analyze and monitor complex data and identify patterns and behavior to detect anomalies and predict risks.
AI algorithms help banks mitigate risks much better and faster, making it easier to prevent fraud and cybersecurity risks.
2. Machine Learning (ML)
Banks extensively use machine learning algorithms, such as logistics regression, decision trees, and anomaly detection by analyzing existing data to predict potential outcomes or identify risks.
These ML models help banks identify outliers and unusual patterns that indicate potential risks and suspicious activities, preventing risks of fraud.
3. Natural Language Processing (NLP)
NLP in banking helps banks analyze structured or unstructured data from multiple sources, such as news, articles, customer communications, and social media, to assess user sentiments, monitor regulatory changes, and identify emerging threats and risks.
Analyzing this data also helps banks enhance their overall application processing speed and onboarding, improving customer experience.
4. Deep Learning
Deep learning is a machine learning model that uses neural networks to learn from huge data sets. Deep learning algorithms, especially neural networks, handle complex risk management and modeling tasks, including fraud detection, credit scoring, and cybersecurity threat detection.
When it comes to the banking sector, banks and financial institutions especially employ deep learning to ensure the accurate calculation of a user's credit score when applying for a credit card loan and to identify and predict potential risks that may impact the user’s creditworthiness.
5. Big Data and Analytics
Big data analytics technology provides banks and financial institutions with the capabilities and infrastructure to analyze a huge volume of structured and unstructured data.
By enabling data processing, aggregation, storage, scalability, and real-time analytics, big data helps banks enhance their risk management capabilities, enabling them to make timely decisions and mitigate risks more effectively.
6. Robotics Process Automation (RPA)
RPA in risk management automates rule-based and repetitive tasks, such as data validation, data entry, and report generation to streamline processes and reduce risks of errors.
This helps banks improve the systems’ accuracy, credibility, efficiency, and productivity.
Emerging Applications of AI in Risk Management for Banks
1. Fraud Detection
Fraud detection is one of the most critical applications of AI risk management in banking. Fraudsters and cybercriminals carry out several malicious, fraudulent activities to gain access to user accounts and steal money. These can include fraudulent credit loan applications, account takeover, money laundering, and overpayment scams.
AI and ML algorithms can easily identify these fraudulent activities by analyzing a large volume of data and detecting anomalies that indicate suspicious behavior. By leveraging real-time data analysis, AI and ML-based algorithms help banks stop fraudulent activities and transactions, minimize losses, and maintain the integrity of their operational and lending profiles.
2. Customer Risk Profiling
Artificial Intelligence-based customer risk profiling helps banks analyze customer data, including customer transactions, behavioral patterns, and credit history, to assess every customer’s risk level—which wouldn’t have been feasible and as accurate when done manually.
Thus, machine learning algorithms allow banks to identify high-risk profile customers with unusual transaction patterns, overarching payments, or other suspicious activities to implement risk mitigation measures such as account monitoring or enhanced due diligence.
3. Regulatory Compliance Management
Complying with standard regulations is extremely important for banks and financial institutions to operate efficiently within ethical and legal boundaries and maintain the integrity of financial systems.
While complying with these regulations can get complex as it involves analyzing customer data and transaction records with accurate documentation and reporting, AI and ML algorithms automate these processes to ensure accuracy.
AI technologies automate Know Your Customer (KYC) and Anti-Money Laundering (AML) processes to ensure compliance with financial regulations, anti-fraud laws, and customer protection mandates, eliminating compliance risks and penalties for banks.
4. Operational Risk Management
AI algorithms help banks predict their operational risk efficiency by identifying and mitigating operational risks, including human errors, IT failures, and compliance violations.
AI proactively addresses operational risks by analyzing past patterns and historical data, operational metrics, and incident reports to predict potential incidents, improve operational efficiency, reduce costs, and enhance customer experience.
5. Insider Threat Prevention
Since AI continuously monitors the users and their behavior, it helps detect and identify early signs of insider trading, rogue trading, fraud, market manipulation, data theft, and other types of financial crime.
This helps banks and financial institutions identify and mitigate fraud and potential insider threat risks, maintaining the banks’ integrity and credibility.
Future of AI risk Management in Banking
According to a McKinsey report, the future of bank risk management is expected to change significantly by 2025, as a result of facing new demands and requirements in the market.
The risk operations for banks in 2025 are more likely to have broader responsibilities toward better analytics and greater collaboration. In fact, the report states that the future risk management model will see a massive shift towards new models and analytics, such as machine learning.
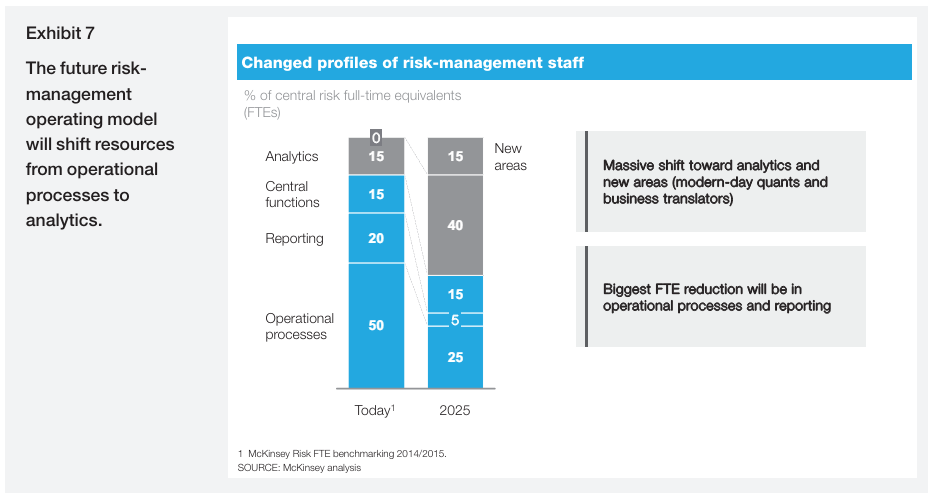
Thus, it’s safe to say that AI and ML hold significant importance and implementation in the future of bank risk management. Future advancements in risk management may include sophisticated machine learning models, such as reinforcement learning and deep learning, to handle complex risk scenarios.
Moreover, when it comes to enhanced collaboration, banks will collaborate with technology vendors, fintech startups, and regulatory authorities to employ AI-powered collaborative risk management platforms to facilitate data sharing and risk intelligence exchange—enabling better and faster risk mitigation.
How can Arya AI Help with Risk Management?
AI is definitely the future of bank risk management, and we at Arya AI provide all the necessary and crucial APIs that you can integrate seamlessly into your existing systems to prevent fraud risks and strengthen security and risk management systems.
A few of these APIs are:
- The bank statement analyser API reads transactions from the bank statement to convert its content into an analytical report, providing key metrics and making it easier to detect anomalies.
- The bank statement extraction API extracts the account holder’s information, such as account number, first and last transaction record, and statement duration, to get all the relevant information about a particular user at their fingertips.
- The cheque extraction API facilitates and streamlines the redundant cheque screening process by reading handwritten amounts on the cheque in words and figures, payee name, date, and account number to assess their legitimacy and designate specific confidence scores.
Check out more Arya APIs here.
Conclusion
AI-powered risk management is revolutionizing how banks operate and mitigate risks by enhancing accuracy, adaptability, and efficiency.
By leveraging advanced algorithms and machine learning technologies, banks can proactively identify and prevent complex risks and gain unparalleled capabilities, such as fraud detection and credit risk assessment.
This also helps banks reduce costs, improve accuracy, and enhance customer experience, enabling them to stay ahead of the curve.
AI Arya AI, we understand and address the growing bank and financial risks, providing excellent solutions that help enhance your systems’ accuracy and security.