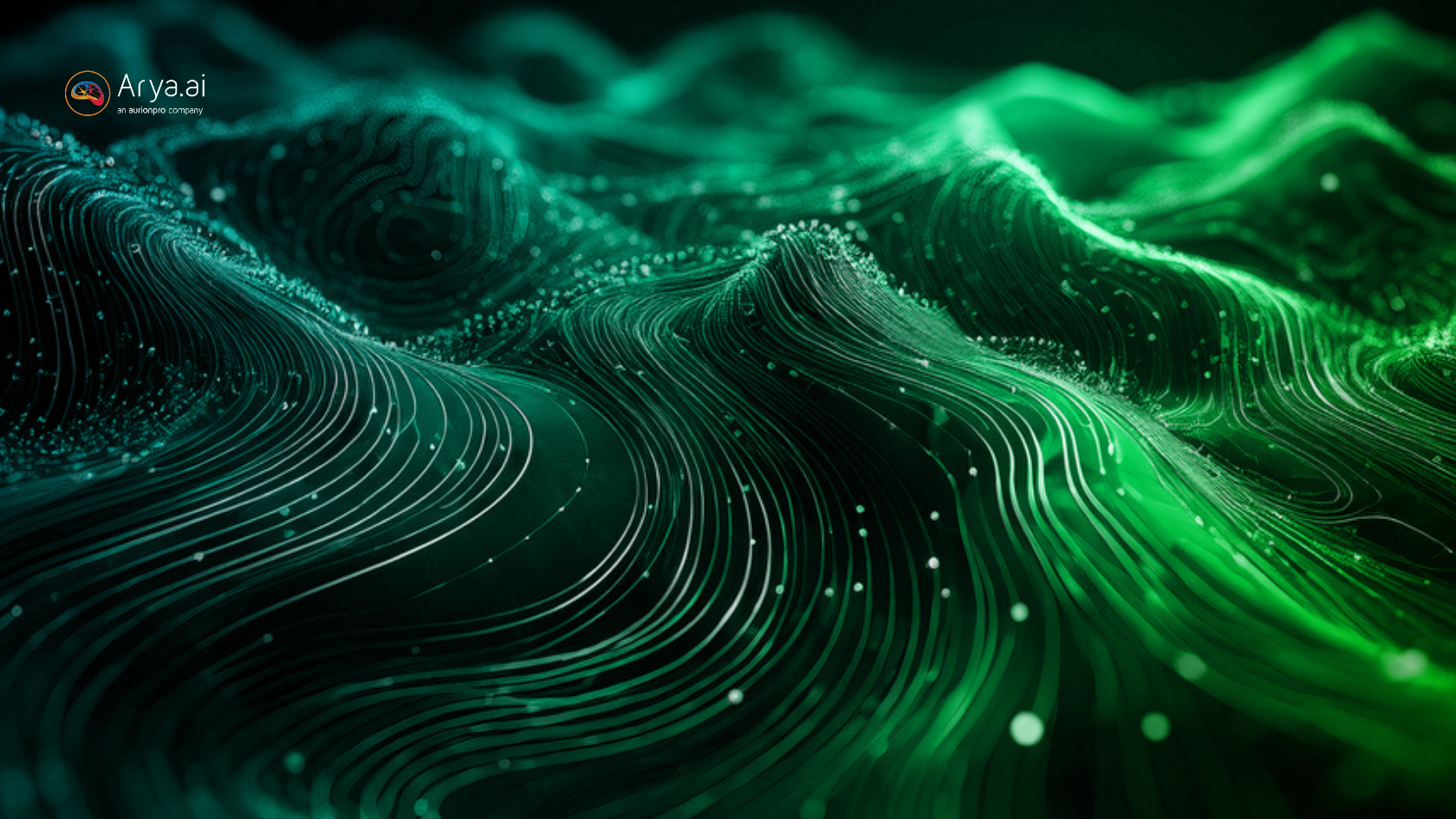
Traditional information retrieval systems in enterprises struggle with siloed data and the sheer volume of information.
LLMs (Large Language Models) are solving this problem for enterprises. They are improving enterprise search by moving it from a simple keyword match system to understanding the meaning and context of the queries and documents/data.
LLM-powered search systems can understand the context of queries with the assistance of natural language understanding. These systems can retrieve the most relevant information from vast corporate repositories and turn enterprises’ “haystacks ” of data into actionable intelligence.
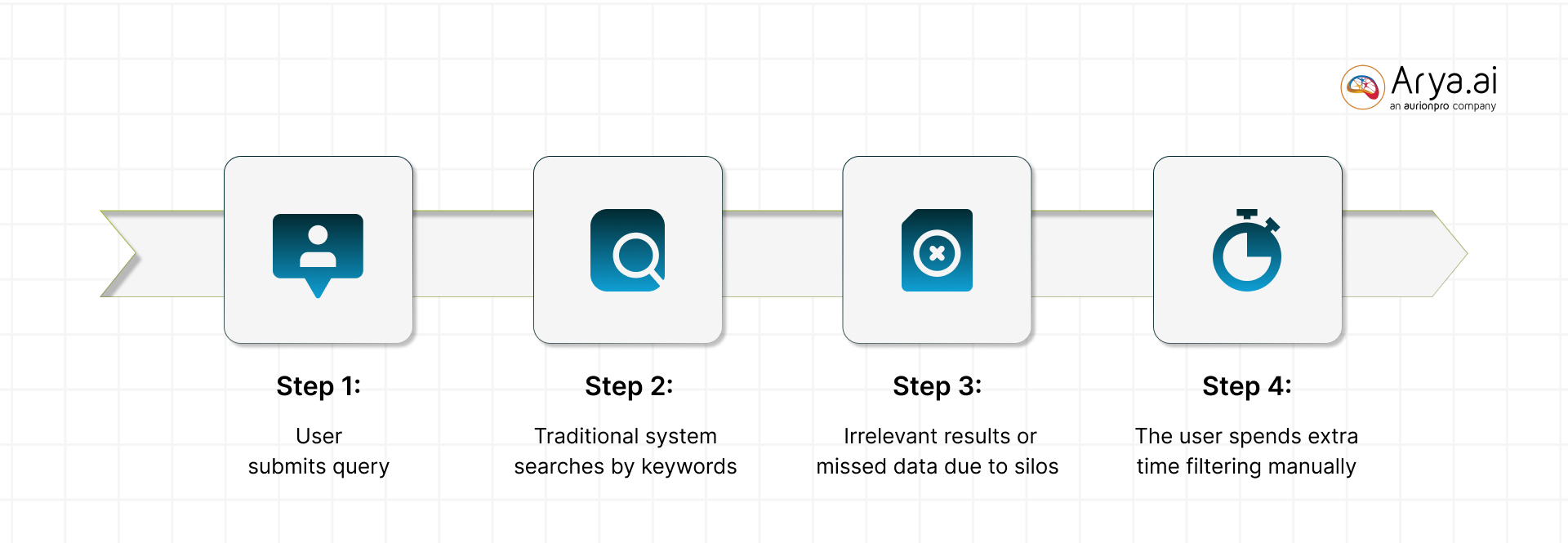
Today, we need an LLM-powered solution for enterprise search because it is fraught with multiple challenges:
- Data silos and fragmentation across multiple, disconnected repositories (databases, CRM platforms, content management systems, cloud storage, etc.)
- Reliance on conventional tools that strictly depend on keyword-based algorithms that deliver useful results only if queries closely match the exact text in documents
- Volume, variety, and velocity of data from multiple channels (emails, support tickets, social media, etc.) existing in structured, semi-structured, and unstructured formats
- Limited context and relevance where platforms struggle with superficially similar terms or to parse context that might change the meaning of a query.
LLMs have the potential to solve these challenges and make information retrieval an absolute breeze for enterprises.
LLMs Enhancing Enterprise Search Capabilities
Let’s look at the areas where LLMs are particularly useful.
Semantic Search
LLMs can perform semantic searches by understanding the intent and context of a query. It goes beyond simple keyword matching, where you consider the query a bag of words. If you search for the term bank,’ the system will pull documents containing the word bank.’ However, based on context, an LLM-powered system could discern whether the user means a financial bank or a river bank and deliver appropriate results.

Ultimately, LLMs’ semantic capabilities considerably improve user intent recognition. They can handle ambiguous or complex queries by considering the surrounding context and likely user needs. Assume a financial analyst searches for “credit risks in emerging markets.” Here, an LLM-aware system can infer that the intent might be to find reports or data about risk factors affecting emerging market credit – even if those documents don’t use the exact phrasing. LLMs close the long-standing semantic gap between users and enterprise content.
Natural Language Queries
Because LLM-powered search understands language well, users can search in plain English (or any natural language) to facilitate a friendly interaction. It’s akin to speaking with a colleague for information. The only difference is that the system has a lot of information at its disposal. For example, a user could ask, “What are our exposure levels to interest rate changes?” and the LLM-powered search might retrieve relevant risk reports, even if those documents use different terminology (e.g., “rate sensitivity analysis”).
Friendly interaction isn’t the only benefit here. For example, if a user asks, "How did our revenue perform last quarter? " An LLM-powered search system would understand the intent behind this query and retrieve the relevant financial reports, summaries, and presentations.
Let’s say the user asked, "What are the key risks in our latest audit report?" Instead of just listing audit files, the system would extract critical insights and summarize key findings, making information retrieval faster and more actionable.
Enterprise Knowledge Graphs & Intelligent Summarization
LLMs can create Enterprise Knowledge Graphs that connect data across multiple sources to extract more profound insights. Instead of merely retrieving documents, LLM-powered search can summarize key findings, extract key entities (names, dates, legal clauses, etc.), and highlight the most critical information.
For instance, if an executive searches for a "summary of recent mergers in the finance industry," an LLM-powered system won’t just list articles—it can generate an executive summary of relevant mergers, including companies involved, deal values, and industry impact.
This ability to synthesize and condense information allows enterprises to move beyond search and toward real-time business intelligence.
Cross-Repository & Multi-Modal Search
Enterprises store data in multiple formats and across disconnected repositories such as emails, CRM systems, customer support tickets, cloud storage, internal reports, and video/audio transcripts.
LLMs break down these data silos by enabling cross-repository search. A single query can retrieve text, images, PDFs, or even insights from video/audio files—all in a unified, easy-to-navigate result set.
For example, an HR manager searching for "employee training feedback" could get sentiment analysis from employee surveys or internal emails discussing training effectiveness. This multimodal retrieval ensures enterprises get holistic insights rather than fragmented, incomplete results.
Why Bother with an LLM-Powered Search in Financial Institutions?
If we have an existing enterprise search system, why bother with powering with LLMs? Financial organizations deal with enormous volumes of information, from regulations and research reports to customer communications. The volume of data alone makes FIs a prime beneficiary of LLM-enhanced search, where it could be useful across:
Risk Analysis and Fraud Detection
These systems can better detect risks and fraudulent activities by searching through and analyzing vast datasets (both structured and unstructured). They can comb through transaction logs, emails, and customer communications to identify suspicious patterns or anomalies that indicate fraud. They can even process free-form text (like payment memos or support call transcripts) to catch clues of fraud that traditional systems might miss.
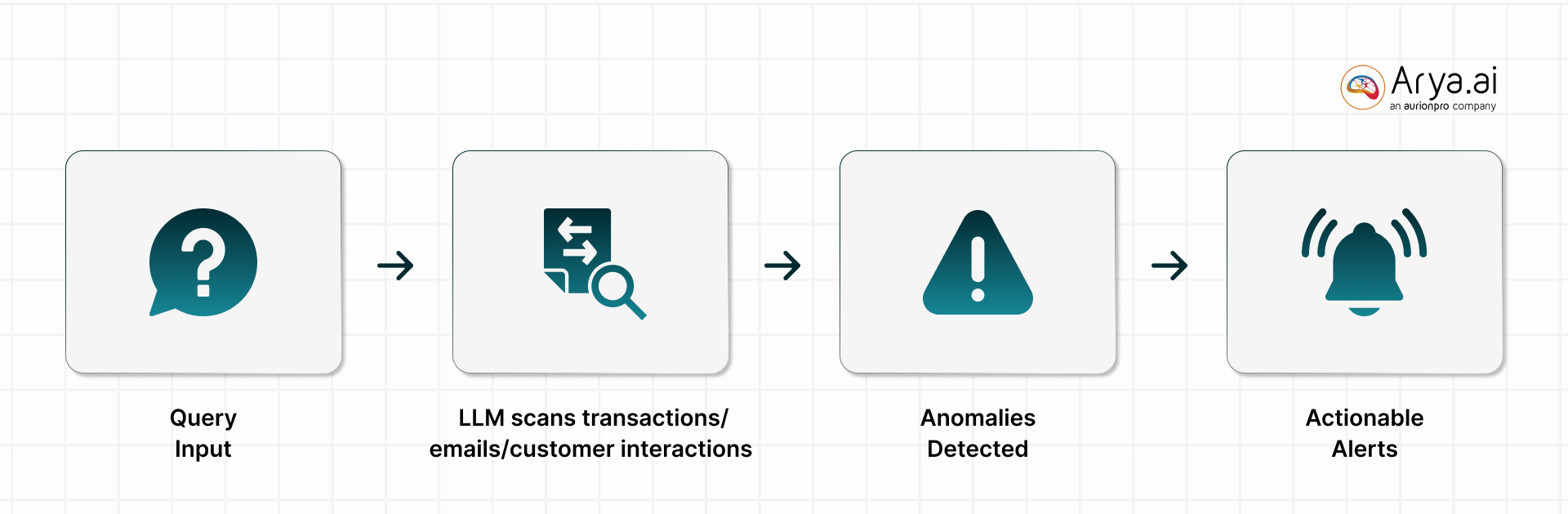
On the risk analysis side, financial LLMs enhance how analysts retrieve and digest risk-related information. Consider credit risk assessment: An LLM can quickly scan a borrower’s financial statements, credit history, news about the borrower’s industry, and even social media sentiment to provide a holistic risk profile.
Internally, risk officers can query an LLM-powered system with questions like “What is our exposure to Southeast Asian market volatility?” and get synthesized answers based on research reports, market data, and internal risk models.
Compliance and Regulatory Intelligence
Compliance teams must constantly search through laws/regulations (including AI regulations) and internal policies to ensure the firm meets all the requirements. LLMs significantly streamline these compliance and regulatory searches. An LLM can interpret complex regulatory text and map it to a firm’s data and policies, acting like an always-alert compliance analyst.

For example, given a query about a new anti-money laundering rule, an LLM-powered system can retrieve the relevant clause from thousands of regulations pages and surface related internal guidelines or past compliance reports. These models excel at sifting through dense legal jargon to find the specific requirements or exceptions that matter.
Market Trend Analysis
Maintaining market trends is paramount in capital markets and investment management. LLMs assist by enabling analysts to efficiently search news feeds, research databases, earnings call transcripts, and social media efficiently for signals and insights.
A financial analyst can ask an LLM-powered system a question like, “Summarize the latest trends in the renewable energy sector”, and receive a concise overview from numerous reports and articles.

They also excel at sentiment analysis and thematic search: An LLM can scan hundreds of news articles or social media posts about a company and tell the user whether sentiment is trending positive or negative and why. For more quantitative analysis, LLMs can be used alongside vector databases to find similar past market scenarios (e.g., “find periods analogous to the 2020 pandemic shock”) by searching through historical data descriptions.
Market Research
Another powerful use is in research. Financial researchers often have specific questions, like “How did commodity prices react after the last Fed rate hike?” Traditionally, they would manually find reports or charts. An LLM, especially one trained in financial corpora, can directly answer by retrieving the data from internal knowledge bases or external sources. Bloomberg GPT, a domain-specific LLM, was designed to support such tasks – it’s trained on a wide range of financial data to perform NLP tasks like news classification and Q&A in finance.
This means it can help a user quickly classify if the news is about earnings, M&A, or regulatory issues or answer a query about market indicators. Integrating LLMs into market analysis platforms allows traders and portfolio managers to get insights faster, leading to quicker decision cycles.
They can query the AI for analysis that would take human hours—like summarizing all central bank statements from the last month—and use that information to inform trading strategies. By augmenting human analysts’ search and summarization capabilities, LLMs help financial institutions react to market trends more efficiently and even anticipate them by uncovering hidden patterns and connections in the data
Customer Support
Let’s break it down. Firstly, on the customer side, LLM-powered chatbots’ ability to retrieve details behind the scenes is unparalleled. So when a customer asks a complex question, like “How do I dispute a charge on my credit card from overseas?” the chatbot’s LLM component can pull relevant information from the bank’s knowledge base or policy documents to craft a helpful answer.
These intelligent assistants can handle account inquiries, explain financial products, or guide users through procedures with human-like clarity. For instance, banks have implemented LLM-based virtual agents that understand customers' intent and retrieve the exact solution from thousands of possible help articles. The result is accurate, personalized responses without long hold times or escalations. This improves customer satisfaction by resolving issues quickly and consistently.
Knowledge Management
For internal knowledge management, an LLM-powered search ensures that employees can easily find and use collective knowledge within the organization. Financial institutions often have vast repositories of internal documents—research reports, procedural manuals, training materials, and emails—that have historically been hard to navigate. LLMs change that by allowing staff to query these troves in plain language and get pinpoint answers.
To understand this, we can look at the internal AI assistant that Morgan Stanley uses for its financial advisors. The firm enabled advisors to query an internal knowledge base of thousands of research documents and get instant answers, summaries, or references.
Advisors can ask, “What did our analyst say about tech sector earnings this quarter?” the LLM will retrieve the relevant commentary from research reports, drastically reducing search time. This internal chatbot (dubbed the Morgan Stanley Assistant) became immensely popular, with 98% of advisory teams using it regularly for information retrieval.
Internal Document Search and Research Assistance
In research-driven financial roles (like equity research, investment analysis, or strategy), professionals spend a lot of time searching for information across internal and external sources. LLMs supercharge internal document search and research by combining comprehensive indexing with language understanding.
For example, a query like “internal memo from last year about LIBOR transition impacts” would have been a headache to find in a large bank’s archives. However, an LLM-based search can instantly pull up the exact memo or even quote the section discussing LIBOR since it understands what the query is asking for and the content of each document.
This was essentially what Morgan Stanley’s AskResearch tool did for its research division. By asking a question, the analyst was directed to the precise part of a report that contained the answer rather than wading through irrelevant text.
Additionally, LLMs can help generate first drafts or outlines for research write-ups by pulling facts from various sources (under analyst supervision). Bloomberg’s 50-billion parameter model, BloombergGPT, was designed to assist with these tasks—ingesting news, filings, and research and aiding in question answering and report generation in the financial domain.
Summing Up
The finance sector operates in a sensitive environment. To implement such a system, FIs face various risks and challenges. LLMs require large amounts of data to be effective, which means a bank or insurance company must feed confidential information.
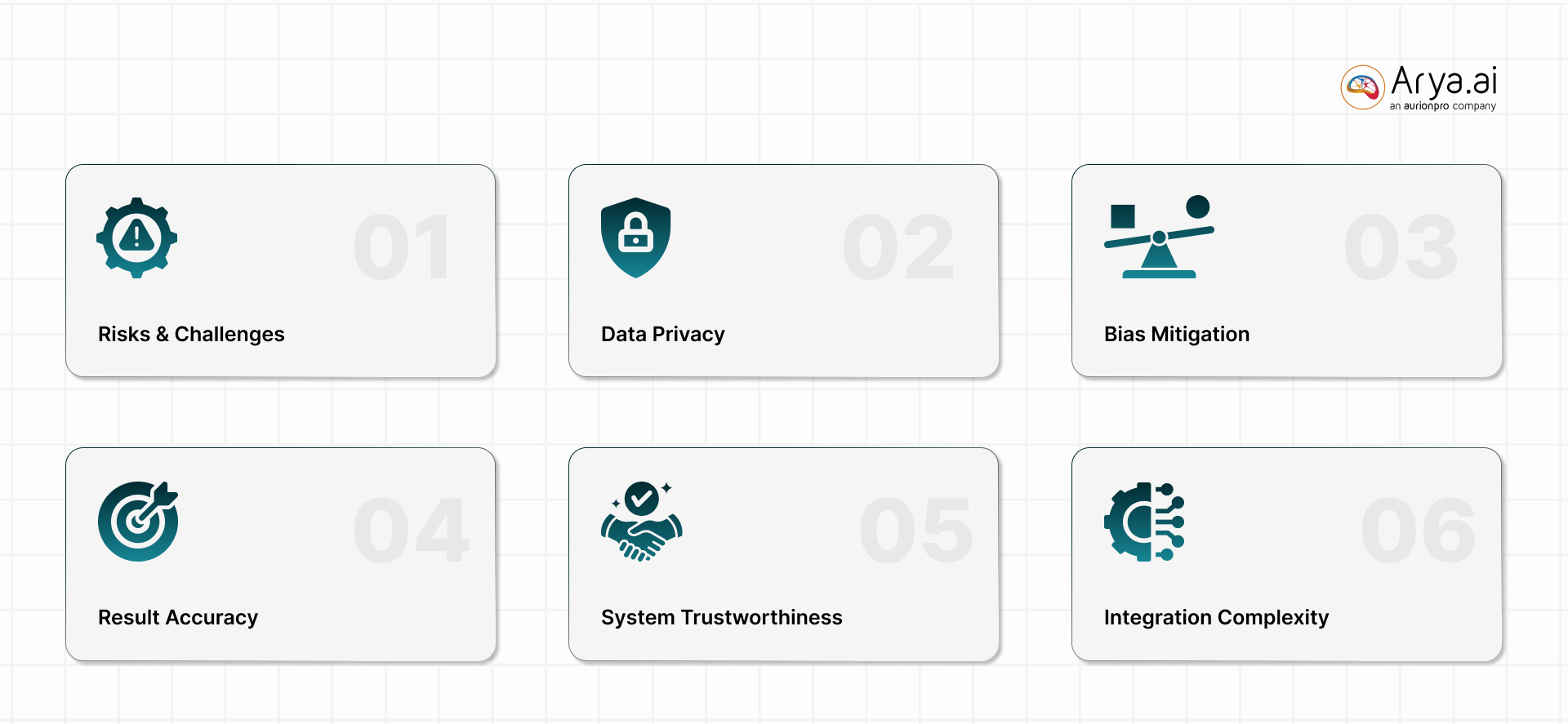
Care must be taken to protect this data during model training and daily query processing. Biar and fairness output, accuracy, and trustworthiness are other critical challenges. How could a financial analyst unquestioningly trust a system when something incredibly significant is at risk? The good thing is that such challenges can be overcome with some additional validation steps.
Ultimately, deploying LLM-powered enterprise search requires a responsible AI approach. Attention to data privacy, bias mitigation, system integration, and result validation is necessary to meet the financial industry's high stakes. However, these challenges can be managed with careful design and governance, allowing the organization to reap the rewards of advanced LLM search capabilities.
Want to harness LLM for your enterprise search? Let's Talk.