
AI is becoming an integral part of the workplace. Most knowledge workers already use Gen AI tools for summarizing reports, writing, and code debugging. But does the enthusiasm sufficiently convey the actual picture? A McKinsey report suggests that only 1% of companies consider AI deployments fully mature and integrated into their workflows.
The problem lies with short-term returns. Long-term benefits are evident, but short-term gains aren’t easily realized or visualized. Even though short-term gains are yet to be transformational, early evidence on ROI is promising: for every $1 invested in AI, businesses on average see $3–$4 in returns, which is expected to grow as this report is two years old.
The spectrum of AI in the workplace is interesting, from opportunities to addressing gaps and from injecting capital to driving real value. We’ll explore this spectrum and learn the substantial benefits, where we lie on the spectrum, how AI is used in the workplace, and more.
Key Benefits of Deploying AI in Business Operations
.jpg)
More Efficiency
AI automates high-volume, repetitive tasks in the workplace and augments human work. The notable productivity improvement drives better efficiency across departments. Customer support agents using generative AI assistance handled 13.8% more inquiries per hour. Similarly, professionals using AI to help draft documents wrote 59% more content per hour, and programmers using AI coding tools completed 2X more work simultaneously.
These boosts in output translate to faster service and higher throughput. The time saved can be reinvested in higher-value activities. If organizations truly integrate AI into their systems, even information retrieval becomes easy through LLM-powered enterprise search systems. Not only does AI help make sense of the increased influx of data, but it also helps leverage it to drive automation.
Substantial Cost Savings
Automating processes and reducing human intervention are inevitable cost savings. If AI automates high-volume back-office tasks, it cuts labor and operational costs. In customer service alone, AI adoption has led to an average 35% reduction in service operation costs while boosting revenue by 32% through better customer experiences.
Banks adopting AI report lower operating expenses through streamlined workflows. Alan Turing Institute. Nearly 9% of all hedge funds use ML to build large statistical models. AI’s potential is increasingly being realized, and the adoption numbers will increase substantially.
Better Decision Making
AI systems can analyze vast datasets at speeds and depths, providing leaders with richer insights for decision-making. Machine learning models excel at detecting patterns and trends in data, improving the accuracy of forecasts for sales projections, demand planning, risk assessments, etc..
In practice, this means decisions can not only be automated but also more data-driven and evidence-based. Whether an algorithm recommends optimal inventory levels or an AI model identifies emerging customer preferences, AI augments human judgment with faster and often more objective analytics. This can lead to better outcomes, such as fewer frictions due to AI-cash flow forecasting.
Better Services and Ample Time for Innovation
AI deployment takes on the onus of maintaining consistency in high-volume, repetitive tasks. It can continue to perform well on routine tasks around the clock, like quality checks or responding to common customer queries. Employees freed from drudgery can concentrate on creative and strategic work, boosting morale and driving innovation.
AI tools can spur innovation and new product ideas by revealing unmet needs in data or enabling rapid prototyping (for example, using generative AI to mock up designs or simulate market responses). Across domains, the fusion of human creativity with AI’s capabilities can lead to novel solutions and improvements that neither could achieve alone.
Challenges with AI in the Workplace
While AI's advantages are compelling, organizations must navigate several trade-offs and challenges when implementing it at work.
The Ethical and Bias Challenge
AI systems can inadvertently introduce or amplify biases present in training data, raising fairness issues in sensitive applications like hiring or lending. For example, an AI resume screener trained on a company’s past hiring could learn gender or racial biases and unfairly rank candidates. Likewise, biased algorithms in credit scoring might systematically favor or disfavor certain groups, echoing historical inequities. Such outcomes are unjust and can lead to legal and reputational risks.
Data privacy is another ethical dimension, especially when AI thrives on big datasets. This is even critical for financial institutions bound by complex AI regulations. Because of the complex architecture of data and information, many organizations are trying to opt for edge AI in finance, which ensures data does not leave the premises.
The Workforce Challenge
Substantial efficiency gains from AI automation put certain tasks at risk, including some entry-level jobs. One UK report estimated that AI could displace 1–3 million jobs in the UK over the coming years (with annual peaks of up to 275,000 job losses during transitions). However, AI can potentially transform jobs more than outright destroy them.
Routine tasks get automated, but new tasks and roles emerge, requiring employees to upskill and work alongside AI. “It won’t be a zero-sum game… certain tasks will become automated, but the largest amount of change will be in the evolution of roles.” This makes workforce adaptation critical. Companies must invest in reskilling programs to help employees transition to roles AI cannot fill and leverage AI to complete those tasks.
The Implementation Challenge
Deploying AI at scale isn’t just a plug-and-play proposition. It comes with practical hurdles. Data quality and integration are often significant challenges – AI needs large, clean datasets, which many firms struggle to provide. There are also technical and financial costs: developing AI capabilities or buying enterprise AI solutions can be expensive, and ROI may take time if implementation is poor.
Change management is essential. And in regulated industries, there’s a tension between innovation and compliance – e.g., strict regulations might limit how banks can use AI for lending. All these factors mean that achieving AI’s promise requires thoughtful strategy and execution, not just technology for technology’s sake.
Rundown of Application of AI in the Workplace
Despite these challenges, the trajectory is clear: organizations that navigate ethical and workforce issues responsibly stand to reap huge benefits, while those that ignore the pitfalls risk backlash or failure. Next, we examine how specific business functions leverage AI and which areas are likely to remain human-driven due to their nature.
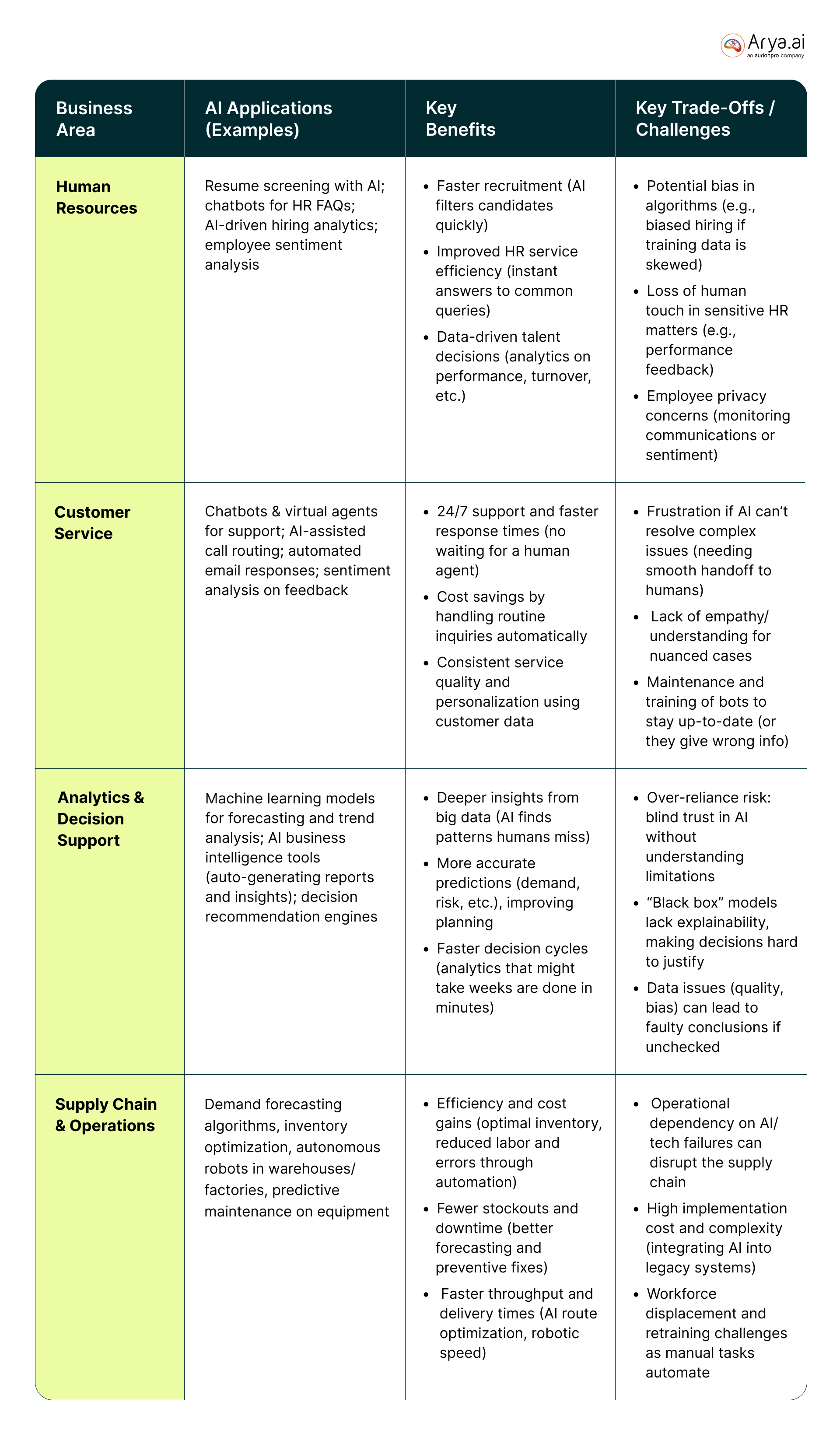
How AI is Helping Organizations
Agentic AI Takes Decisions Autonomously
Agentic AI systems are designed to act autonomously within defined parameters, making decisions with minimal human intervention. These systems learn from their operational environment and adjust their actions based on real-time feedback, significantly enhancing efficiency.
Multimodal AI Processes Text, Audio, Video, and Code
Multimodal systems are also increasingly being implemented across enterprises. These systems process diverse forms of data (text, audio, video, and code) into a unified framework. By bridging these various media, multimodal AI enables more enriched interactions and comprehensive analyses.
Adaptive AI Learns Continuously
Adaptive AI systems take personalization and continuous improvement a step further by constantly learning from new data and evolving to meet specific business needs. Unlike traditional models that may require periodic updates, adaptive AI is engineered to adjust its algorithms on the fly, ensuring that insights and recommendations remain current and relevant. This adaptability is evident in applications such as predictive maintenance, where AI anticipates equipment failures by recognizing subtle performance metrics changes, and dynamic inventory management, where supply levels are adjusted based on real-time consumer behavior.
Role of AI in Financial Operations
The finance industry deals with massive data volumes and values speed and accuracy, making it a fertile ground for AI. At the same time, it also deals with complex regulations. However, financial institutions leverage AI responsibly across various functions and reshape their workflows, risks, and competitive stance.
.jpg)
AI in Fraud Detection and Security
Banks and payment companies leverage AI to detect fraudulent transactions and cybersecurity threats far more effectively than manual methods. Machine learning models monitor account activity in real time and flag anomalies that suggest fraud.
These models continuously learn from new fraud cases to improve their accuracy. The result has been a dramatic improvement in fraud prevention. The U.S. Treasury, for instance, reported that incorporating machine learning into its cheque fraud detection process helped recover over $1 billion in fraudulent payments in one year. At Arya.ai, we have also helped a partner bank process 20+ cheques in a second, which saved over 55% in processing time.
Credit Scoring and Lending
Traditionally, determining creditworthiness (for loans, credit cards, mortgages) relied on relatively limited data (credit scores, income, debt ratios) and often manual underwriting for complex cases. AI has revolutionized this by enabling credit scoring models that use a wide array of data and find nuanced patterns indicative of risk.
Large banks have begun integrating AI into their lending platforms to automate the underwriting process. For instance, ICICI Bank (India) has integrated AI into its lending platform, streamlining the loan approval process and drastically cutting down processing times. Moreover, the State Bank of India employs predictive analytics to identify and prevent fraudulent transactions, showcasing AI’s critical role in strengthening financial security.
The flip side of AI-driven lending is the concern about fairness and transparency. If a human denies a loan, they can explain the reasons. Explaining the decision can be difficult if an AI black-box model denies a loan. Yet, regulations (like the U.S. Equal Credit Opportunity Act) require giving applicants an explanation. This has pushed the development of explainable AI in credit scoring.
Customer Personalization and Service in Finance
Banks and insurers have vast customer data (transaction histories, web/app behavior, demographics), and AI is helping them use it to tailor products and services. In retail banking, AI analyzes customer data to personalize marketing. For example, identifying which customers are likely to need a home loan and proactively offering one at the right moment. AI can power personal financial management tools that give customers insights into their spending, nudge them to save, or alert them of unusual activity.
Chatbots, like Bank of America’s “Erica” or Capital One’s “Eno,” serve banking customers by answering questions about their accounts, helping them make transactions, or providing credit score updates. These AI assistants improve convenience and handle millions of queries that would otherwise tie up human agents.
In insurance, AI is used for more personalized pricing, like analyzing a customer’s behavior ( driving data from telematics devices) to offer usage-based insurance pricing tailored to that individual’s risk profile. This granularity can benefit safe customers with lower premiums and incentivize good behavior (e.g. safe driving), while insurers better manage risk selection.
The Human Advantage: Work That Still Relies on Human Ingenuity
The “human advantage” areas involve creativity, empathy, and higher-order thinking—areas where human ingenuity is essential. AI will continue to advance and may encroach a bit more into what we consider creative or emotive tasks (for example, AI can write a passable news article or compose music in a certain style).
But even when AI contributes, human oversight and input are needed to ensure relevance, quality, and ethicality. Rather than viewing it as humans versus machines, many experts now talk about “collaboration between humans and AI.” The most successful organizations will be those that pair each with their strengths. It could be using AI for pattern processing and automation, humans for ingenuity and interpersonal connection. In the foreseeable future, tasks like devising a corporate strategy, crafting a compelling story, or nurturing a team’s culture remain human-led endeavors, albeit supported by AI-generated data and suggestions.
Conclusion
AI’s deployment in the workplace will lead to substantial gains in productivity and innovation, but it will also change how organizations operate and employees perform their roles.
Presently, companies are capitalizing on AI to automate drudgery, illuminate patterns in big data, and respond with agility to business needs. Across functions like HR, customer service, analytics, and supply chain, AI is proving its value by handling high-volume tasks and providing decision support, thereby allowing humans to focus on higher-level work.
Early adopters in sectors such as finance demonstrate how AI can bolster fraud prevention, speed up lending, and sharpen competitive edge when integrated thoughtfully. Yet, these advances come with important trade-offs. Ethical considerations like algorithmic bias, privacy, and transparency have moved to the forefront, requiring organizations to implement robust oversight and inclusive design of AI systems.
Organizations that strike this balance are likely to accomplish the right outcomes. We at Arya.ai are building enterprise-grade AI solutions. These production-ready solutions can be deployed on-premise or in the cloud, and, depending on your requirements, we can even build bespoke solutions.
Talk to us if you want to integrate secure AI solutions into your enterprise.