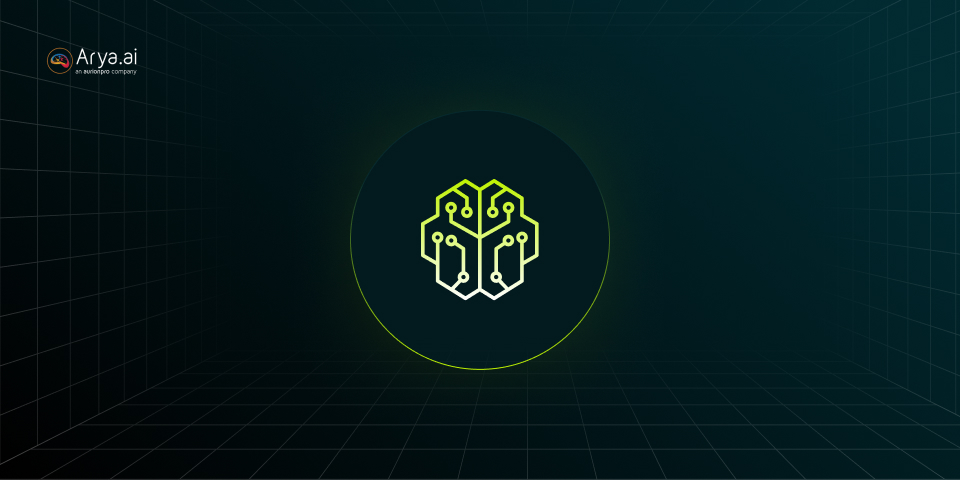
Artificial intelligence is reshaping banking, financial services, and insurance (BFSI) by automating tasks, improving decisions, and enhancing customer experiences. In fact, AI could reduce operational costs for financial institutions by up to 22% (an estimated $1 trillion savings by 2030). No wonder 76% of banking executives say AI is crucial for differentiation.
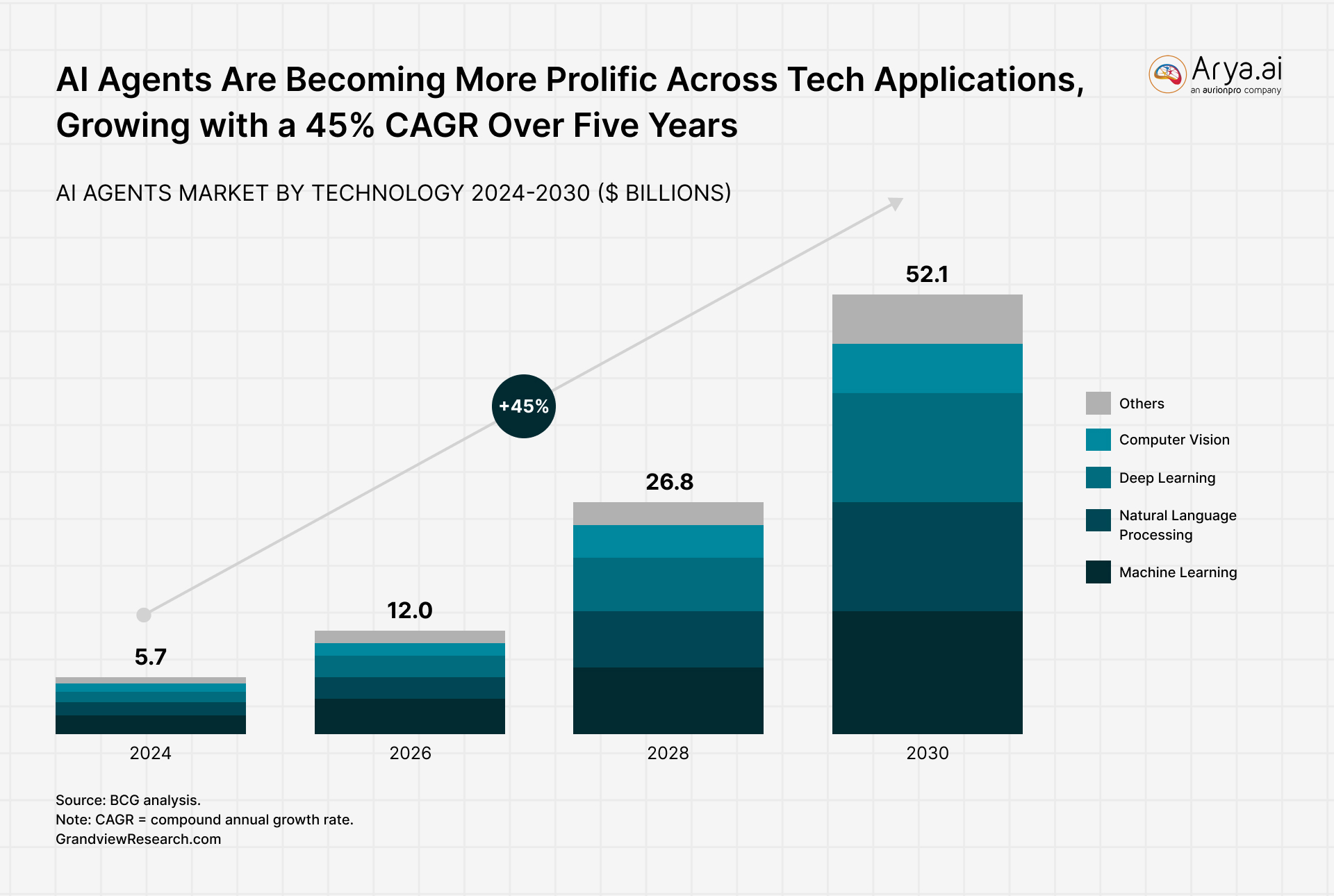
As BFSI firms embrace AI Agents – intelligent software that autonomously assists or acts on behalf of humans – they face a critical decision: build in-house or buy off-the-shelf? This analysis examines key AI use cases in BFSI and evaluates the cost-benefit, risks, and implementation challenges of building vs. buying AI solutions, drawing on industry reports and real examples. The goal is to help decision-makers chart an optimal AI adoption strategy.
What Are AI Agents, and Why Are They Critical for BFSI?
AI agents are intelligent software solutions that perform tasks autonomously or in collaboration with humans. They can automate repetitive processes, streamline decision-making, and enhance customer experiences by operating independently or augmenting human efforts. Their ability to swiftly analyze vast data sets and learn from interactions makes them ideal for BFSI, where speed, accuracy, and scalability are critical.
Recent advancements in AI, particularly Small Language Models (SLMs) and Large Language Models (LLMs), have expanded the potential of AI agents significantly. SLMs (smaller, domain-specific models) provide focused, cost-effective solutions suitable for targeted use cases, such as processing specialized financial or insurance documentation. In contrast, LLMs, like GPT-4, offer sophisticated capabilities for natural language understanding and generation, powering applications such as advanced customer chatbots, automated financial reporting, and personalized investment advice.
Build vs Buy (AI Agents): AI Use Cases Across BFSI Functions
AI agents can drive automation and insights across front-office and back-office functions in BFSI. Here are the major areas where BFSI organizations are leveraging AI:
1. Customer Service & Virtual Assistants
AI chatbots handle routine banking and insurance queries, improving response times and reducing costs. Bank of America’s “Erica” has managed 2B+ interactions for 42M customers. Adoption is growing—only 12% of banks use AI for customer service today, but ~50% plan to implement it soon.
2. Fraud Detection & Prevention
AI detects fraud in real-time by analyzing transaction patterns, reducing false positives, and improving accuracy. 75% of financial firms using AI report a 10–20% drop in fraud cases. Continuous model updates are crucial to maintaining accuracy.
3. Credit Risk Assessment & Underwriting
\With AI Agents in place, it enhances loan approvals by analyzing spending behavior and alternative data, enabling faster, more precise credit scoring. Insurers use AI for pricing policies, with firms like Daido Life incorporating AI-driven risk models that offer transparency alongside speed.
4. Regulatory Compliance & Reporting
Automating compliance tasks like AML monitoring and regulatory reporting, cutting false positives and manual workload. J.P. Morgan’s COiN platform processes 12K+ contracts annually, saving 360K hours of legal review.
5. Investment Advisory & Wealth Management
AI powers robo-advisors, market analytics, and financial product recommendations, improving investment personalization. Automated portfolio rebalancing helps wealth managers serve more clients at lower costs. Ethical AI use is key to aligning recommendations with client interests.
6. Claims Processing (Insurance)
AI Agents can accelerate claims handling by automating validation, fraud checks, and approvals. Insurers using AI process simple claims in minutes, reducing errors and boosting efficiency. Automated claim updates cut status-related customer calls by 40%.

Build vs Buy (AI Agents): Cost-Benefit Analysis
When adopting AI agents, BFSI organizations must compare the costs and benefits of developing in-house (build) versus purchasing third-party solutions (buy). Both approaches have financial implications and ROI considerations:
Analysis#1: Up-Front and Ongoing Costs
Building an AI solution in-house requires significant up-front investment – hiring or training data scientists, purchasing infrastructure or cloud resources, and spending months (or years) in R&D. Large banks that embark on in-house AI projects often face high costs and risk overruns; many internal tech builds end up “exceeding budget and underdelivering” on expectations.
On the other hand, buying an off-the-shelf AI product typically involves licensing or subscription fees. The initial cost can be lower since the heavy development is already done, but ongoing vendor fees accumulate. Over time, a well-built in-house solution might be cheaper to run (no licensing markup) – but only if the institution can achieve sufficient scale and usage.
A summary of cost considerations is provided below:

Analysis#2: Total Cost of Ownership (TCO)
Over a 5+ year period can tilt the balance. If a bank has the capability to build and maintain an AI agent that becomes core to operations, the higher upfront cost may be justified by savings later (avoiding perpetual license fees). For instance, an in-house AI fraud system might pay for itself after a few years of not paying a vendor, if it performs equally well.
However, this assumes the project succeeds; in-house efforts carry delivery risk. In contrast, purchasing a proven solution means the bank starts saving or earning benefits sooner – a quicker payback period. According to industry surveys, banks that invested strongly in AI are already seeing payoffs: 68% of finance departments report significant ROI and tangible benefits from AI investments in recent years. The quicker deployment from buying can help achieve those gains faster, albeit at the cost of ongoing payments.
It’s also worth noting the opportunity cost of time. A midsize bank could spend two years building a custom AI personalization engine, while competitors buy a ready solution and deploy it in 3 months. Those two years of lost benefits are an implicit cost of building. Many banks, especially smaller ones, find that partnering with AI providers yields value “at a fraction of the cost” and time compared to in-house development. This speed-to-market advantage can outweigh the allure of owning a custom system.
Analysis#3: Effectiveness and Performance
A world-class AI model can drive huge gains – e.g., a top-tier fraud AI might catch significantly more fraud or save millions more than a basic model. If such a model is available via a vendor, buying might deliver greater benefits than a rudimentary model a bank’s small team can build. Banks should honestly assess whether their in-house team can match the performance of specialized AI firms. As one analysis noted, specialist AI vendors continuously invest in refining models and attract top talent, often delivering superior technology than what most banks can achieve internally. If the quality gap is large, buying yields higher ROI through better outcomes (e.g. higher fraud detection rates, better customer satisfaction scores from a chatbot, etc.).
Analysis#4: ROI Over time
This is ultimately a function of initial costs, ongoing costs, and the magnitude of benefits (cost savings, new revenue, improved retention, etc.). Industry-wide, AI is expected to boost profits substantially – a Citigroup report projected AI could propel banking industry profits by $2 trillion by 2028 (a 9% increase).
Achieving such ROI requires successful implementation. Whether build or buy, financial institutions should model the business case: estimate the investment, the expected efficiency gains or revenue lift, and how quickly those accumulate. Many organizations choose a hybrid approach: buy an AI platform or components and then build custom enhancements on top.
This can reduce time to value while still allowing differentiation. For example, a bank might license a proven AI risk analysis engine, and then have internal data scientists tweak the models with proprietary data to get an edge – effectively combining buy and build.
Build vs. Buy (AI Agents): Risk Assessment
1. Data Security & Privacy
Financial data is highly sensitive. In-house AI keeps data within internal controls, reducing third-party exposure. Vendor AI, especially cloud-based, introduces risks—20% of 2022 breaches were linked to third-party providers. Institutions must vet vendors, ensure compliance (e.g., GDPR), and define clear contract terms for encryption, breach protocols, and data residency. While building in-house centralizes security responsibility, both approaches require robust cybersecurity measures.
2. Regulatory Compliance & Model Risk
BFSI firms are accountable for AI decisions, regardless of build or buy. Explainability is crucial—black-box models can create compliance risks. In-house AI allows more visibility into decision-making, aiding regulatory approvals. Vendor AI may lack transparency, requiring rigorous due diligence on audit logs, explainability features, and regulatory scenario testing. Regulatory bodies expect the same risk management standards for vendor AI as for internal models, meaning AI governance is critical in both approaches.
3. Bias & Ethical Risks
AI bias can lead to unfair decisions in credit, insurance, and customer service. In-house development enables direct bias mitigation through data curation and fairness constraints. Vendor AI requires thorough vetting, but proprietary models may limit bias assessment. BFSI firms remain legally accountable for biased decisions, even if AI is outsourced. Reputational damage from AI errors can be severe, making strong oversight (e.g., human-in-the-loop for high-impact decisions) essential.
4. Vendor Lock-In & Dependency
Third-party AI introduces dependency risks—outages, service discontinuation, or contract constraints can disrupt operations. Switching vendors or moving AI in-house later can be costly. Banks should ensure contingency plans (e.g., data portability, contract exit clauses). In-house builds avoid vendor reliance but depend on key personnel—losing expertise can stall progress. Shifting priorities or budget cuts can also derail internal AI projects, leading to wasted investments. Risk mitigation involves documentation, cross-training, and using open-source frameworks where possible.
5. Data Quality & Integration Risks
AI is only as good as the data it processes. BFSI firms often face siloed, inconsistent data. In-house builds require extensive data unification efforts, while vendor AI may provide pre-built connectors—but integration is rarely seamless. Poor data quality can derail AI projects, leading to inaccurate predictions or failed deployments. Careful data governance, phased rollouts, and continuous monitoring are key to reducing these risks.
Key Takeaway:
Build vs Buy (AI Agents): Implementation Challenges and Deployment Considerations
1. Legacy Systems and Integration
BFSI institutions rely on decades-old core systems with fragmented data. Whether building or buying AI, integration is a major hurdle due to siloed data (customer info, transactions, documents). In-house development requires extensive data engineering and possibly modernizing infrastructure (e.g., cloud migration). Vendors promise “seamless integration,” but APIs and customizations are still needed. Phased rollouts—starting with batch data before real-time deployment—help mitigate risks.
2. Technical Expertise and Talent
AI success depends on data science, engineering, and domain expertise. In-house development demands scarce AI talent—70% of financial firms report shortages. Buying shifts expertise needs to vendor management, but internal teams must still configure, oversee, and trust the AI. IT resistance or lack of agile experience can slow adoption. Change management is key—whether building or buying—to ensure AI adoption by employees (e.g., training call center staff to work with chatbots). Maintenance adds complexity: in-house solutions require ongoing talent, while vendor reliance risks slower response times. Institutions should assess internal AI maturity before choosing a path.
3. Time to Deploy and Scalability
Speed matters—AI projects in BFSI often take months due to risk reviews. Buying a pre-built solution accelerates deployment (e.g., AI underwriting engines can launch in weeks vs. a year for custom builds). Vendor solutions also solve scaling issues upfront but aren’t always plug-and-play. Due diligence is needed to ensure they handle real-world volumes (e.g., chatbots managing 50k queries/hour). Cloud-native builds offer scalability but require in-house expertise. AI maintenance is continuous—models need monitoring, retraining, and updates. Vendor AI-as-a-service solutions handle updates, but banks must validate changes to avoid conflicts. Buying enables faster deployment; building offers long-term control.
4. Regulatory and Organizational Hurdles
AI in BFSI faces strict compliance checks—any customer-facing or decision-impacting AI requires risk committee approvals. Vendor solutions don’t eliminate governance needs and may add vendor certification steps. Regulatory uncertainty leads to cautious rollouts. AI projects also suffer from “pilot purgatory” without clear executive mandates. AI Centers of Excellence help streamline efforts. User training is critical—advisors, claim adjusters, and risk managers must trust AI insights. Employee resistance over job security can hinder adoption; leadership must emphasize AI as an enabler, not a job replacer, showing productivity and service improvements post-AI deployment.
5. Maintenance and Support
Post-launch, AI needs continuous updates. In-house builds require dedicated resources to address bugs, new fraud patterns, and evolving business needs. Vendor AI solutions include support contracts, reducing IT burdens but introducing dependency on vendor SLAs. If AI errors impact operations (e.g., false transaction blocks), internal teams can fix issues faster, while vendors may delay resolutions. Compliance-driven changes (e.g., new explainability requirements) require proactive updates. Vendors deploy broad updates, but banks must validate their impact. Institutions should assess vendors’ responsiveness to BFSI regulatory changes before committing.
Key Takeaway:
Build vs Buy (AI Agents): Industry Insights and Benchmarks
1. AI Adoption & Strategic Trends
AI is mainstream in BFSI, research shows that 72% of finance leaders use it for fraud (64%), risk (64%), investments (57%), and automation (52%). Banks lean towards building for emerging AI-like generative models but buy for mature use cases like fraud detection and chatbots. Large banks (JPMorgan, BofA) invest in custom AI, while mid-sized banks prefer vendor solutions. A mixed strategy—building for differentiation and buying for speed—is common.
2. ROI & Performance
In-house AI has driven major efficiencies: JPMorgan’s COiN saved 360,000 work hours, and BofA’s “Erica” hit 1.5B interactions. Buying also delivers quick wins—community banks using AI-driven insights saw improved customer engagement. AI fraud detection tools cut fraud losses by double digits, while AI-powered personalization increased digital sales by up to 20%.
3. Investment Benchmarks
Large BFSI firms spend ~$22M annually on generative AI, with top-tier banks investing over $100M. AI improves productivity (~20% gains in operations and software development). Industry-wide AI investments could save $1T by 2030. The sector spent $20B on AI solutions in 2023, reflecting strong confidence in AI’s value.
4. Hybrid Case Study
A midsize bank needed AI for personalized customer insights. Instead of building from scratch, they bought an AI platform and customized it with in-house data. This hybrid approach ensured a fast rollout while preserving proprietary differentiation—an increasingly popular strategy.
5. Regulatory Considerations
Regulators stress responsible AI, requiring bias testing, explainability, and oversight. HSBC and Barclays have AI governance committees. Many banks test vendor AI in “shadow mode” before deployment. Smaller firms often rely on vendor compliance assurances, while larger banks build governance frameworks.
Key Takeaway:
Build vs. Buy (AI Agents): Recommendations
- Align AI with Business Goals – Prioritize AI use cases based on impact. Build AI for strategic differentiation (e.g., proprietary trading models), but buy for common needs (e.g., chatbots, fraud detection). Define clear success metrics (ROI, efficiency gains) before choosing an approach.
- Assess Costs & Feasibility – Weigh in-house development costs (talent, time, infrastructure) vs. vendor pricing over 5 years. Conduct pilot tests before committing. Buying may offer advanced features that outweigh the cost of building from scratch.
- Mitigate Risks Early – Establish AI governance (IT, compliance, legal) to oversee risks, bias, and security. Vet vendor credentials, negotiate strong contracts (SLAs, exit clauses), and ensure human oversight in AI decision-making.
- Adopt a Hybrid Approach – Combine build and buy: use vendor AI for speed, then customize or build proprietary layers for differentiation. Co-creation with fintechs is another strategic option.
- Invest in Data & Infrastructure – Strong data governance and scalable IT (cloud, APIs) improve AI success, reducing integration delays. Data quality impacts both build and buy strategies.
- Start Small, Scale Gradually – Begin with pilot projects, prove value, then expand. A phased approach minimizes risk and allows for iterative improvements.
- Monitor & Iterate Continuously – AI is dynamic—track performance, refine models, and update strategies. If an in-house model underperforms, consider switching to a vendor, and vice versa.
Conclusion
AI agents have the power to transform BFSI by enhancing fraud prevention, customer service, and compliance efficiency. The choice between building and buying depends on business priorities, resources, and risk appetite. Building offers greater control and competitive differentiation but requires substantial investment and expertise. Buying accelerates deployment and reduces development risk but may lead to vendor dependency and limited customization.
Most successful firms adopt a hybrid strategy—buying for quick wins while building for long-term differentiation. The key to success lies in effective integration, governance, and continuous optimization. Those who balance AI investment wisely will lead in customer experience, risk management, and operational efficiency, securing a competitive edge in the evolving financial landscape.