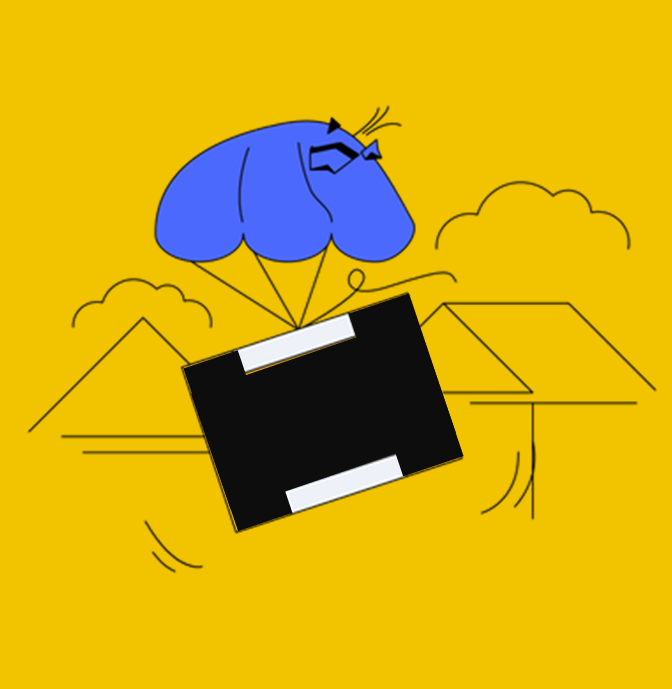
The insurance industry has begun to adopt AI technology in recent years, with many companies automating existing rule sets with machine learning models to bring automation to their existing processes. However, one of the main concerns surrounding AI adoption is the "black box" nature of AI-driven decisions, which makes it difficult for insurers to justify decisions and explain them to their customers, auditors, and regulators.
So, how can a heavily regulated industry, which has always been more inclined to conservatism than innovation, start trusting AI for core processes?
To address this issue, a new field of research called Explainable AI (XAI) has emerged, which aims to help users better understand and interpret the predictions made by AI models. Explainable AI lets us peek inside the AI ‘black box’, to understand the key drivers behind a specific AI decision. Users can more readily interpret the input that is driving the output, and decide on the level of trust on the decision. XAI enables users to see the input that is driving the output, which improves their confidence in the system and allows them to optimize the model based on their expertise. It also promotes transparent communication between insurers and their customers, auditors, and regulators, which is crucial for regulatory compliance and maintaining trust in the decision-making process.
Overall, AI technology has become a survival criterion in the insurance industry, and the adoption of explainable AI provides a way for insurers to enjoy the benefits of AI while remaining compliant and transparent.
Read more on how Explainable AI can help organizations overcome the dilemma between accuracy and explainability.
Related articles

AI Broadcast Series #3: IP & copyright challenges for ‘AI’ solutions and the future of ‘AI’ regulations
See how Arya helps scale
AI in your organization
Learn how to strategise and deploy AI, explore relevant use cases for your team, and get pricing information for Arya.ai products.